A beginner’s guide to interpreting odds ratios, confidence intervals and p-values
Posted on 13th August 2013 by Tim Hicks
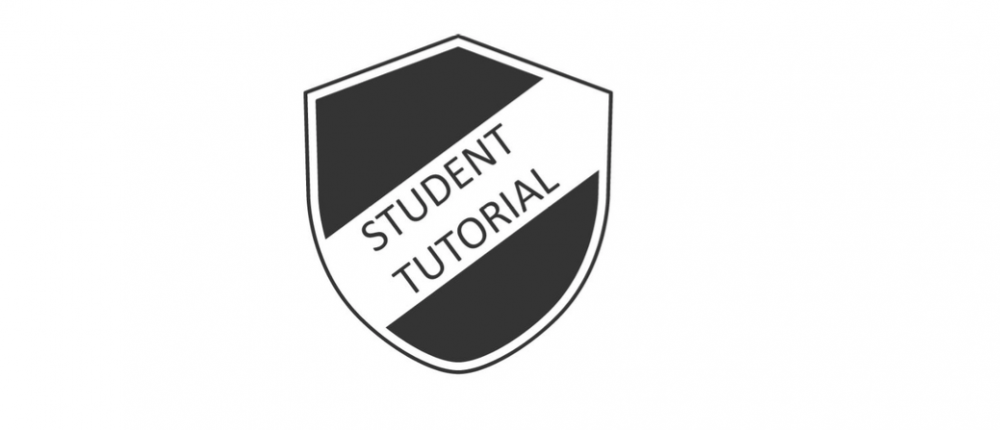
Who is it for?
Students of medicine or from the clinical sciences and professions allied to medicine wanting to enhance their understanding of medical literature they will encounter throughout their careers.
What will I learn and how?
How to interpret odds ratios, confidence intervals and p values with a stepwise progressive approach and a’concept check’ question as each new element is introduced.
How long will it take?
Approximately 20 minutes.
What it is not
A statistical textbook reworded or how to calculate any of these statistics.
Contents:
Introduction
Odds ratio
Confidence interval
P value
Bringing it all together – Real world example
Summary
Self test Answers
Introduction
The first steps in learning to understand and appreciate evidence-based medicine are daunting to say the least, especially when confronted with the myriad of statistics in any paper. This short tutorial aims to introduce healthcare students to the interpretation of some of the most commonly used statistics for reporting the results of medical research.
The scenario for this tutorial is centred around the diagram below, which outlines a fictional parallel two arm randomised controlled trial of a new cholesterol lowering medication against a placebo.
Odds ratio (OR)
An odds ratio is a relative measure of effect, which allows the comparison of the intervention group of a study relative to the comparison or placebo group.
So when researchers calculate an odds ratio they do it like this:
The numerator is the odds in the intervention arm
The denominator is the odds in the control or placebo arm = Odds Ratio (OR)
So if the outcome is the same in both groups the ratio will be 1, which implies there is no difference between the two arms of the study.
However:
If the OR is > 1 the control is better than the intervention.
If the OR is < 1 the intervention is better than the control.
Concept check 1
If the trial comparing SuperStatin to placebo with the outcome of all cause mortality found the following:
Odds of all cause mortality for SuperStatin were 0.4
Odds of all cause mortality for placebo were 0.8
Odds ratio would equal 0.5
So if the trial comparing SuperStatin to placebo stated OR 0.5
What would it mean?
A) The odds of death in the SuperStatin arm are 50% less than in the placebo arm.
B) There is no difference between groups
C) The odds of death in the placebo arm are 50% less than in the SuperStatin arm.
Confidence interval (CI)
The confidence interval indicates the level of uncertainty around the measure of effect (precision of the effect estimate) which in this case is expressed as an OR. Confidence intervals are used because a study recruits only a small sample of the overall population so by having an upper and lower confidence limit we can infer that the true population effect lies between these two points. Most studies report the 95% confidence interval (95%CI).
If the confidence interval crosses 1 (e.g. 95%CI 0.9-1.1) this implies there is no difference between arms of the study.
Concept check 2
So if the trial comparing SuperStatin to placebo stated OR 0.5 95%CI 0.4-0.6
What would it mean?
A) The odds of death in the SuperStatin arm are 50% less than in the placebo arm with the true population effect between 20% and 80%.
B) The odds of death in the SuperStatin arm are 50% less than in the placebo arm with the true population effect between 60% and 40%.
C) The odds of death in the SuperStatin arm are 50% less than in the placebo arm with the true population effect between 60% and up to 10% worse.
P values
P < 0.05 indicates a statistically significant difference between groups. P>0.05 indicates there is not a statistically significant difference between groups.
Concept check 3
So if the trial comparing SuperStatin to placebo stated OR 0.5 95%CI 0.4-0.6 p<0.01
What would it mean?
A) The odds of death in the SuperStatin arm are 50% less than in the placebo arm with the true population effect between 60% and 40%. This result was statistically significant.
B) The odds of death in the SuperStatin arm are 50% less than in the placebo arm with the true population effect between 60% and 40%. This result was not statistically significant.
C) The odds of death in the SuperStatin arm are 50% less than in the placebo arm with the true population effect between 60% and 40%. This result was equivocal.
Bringing it all together – Real world example
A drug company-funded double blind randomised controlled trial evaluated the efficacy of an adenosine receptor antagonist Cangrelor vs Clopidogrel in patients undergoing urgent or elective Percutaneous Coronary Intervention (PCI) who were followed up for specific complications for 48 hrs as outlined in the diagram below (Bhatt et al. 2009).
The results section reported “The rate of the primary efficacy end point was … (adjusted odds ratio with Cangrelor, 0.78; 95% confidence interval [CI], 0.66 to 0.93; P=0.005)
What does this mean?
A) The odds of death, myocardial infarction, ischemia-driven revascularization, or stent thrombosis at 48 hours after randomization in the Cangrelor arm were 22% less than in the Clopidogrel arm with the true population effect between 34% and 7%. This result was not statistically significant.
B) The odds of death, myocardial infarction, ischemia-driven revascularization, or stent thrombosis at 48 hours after randomization in the Cangrelor arm were 34% less than in the Clopidogrel arm with the true population effect between 7% and 22%. This result was statistically significant.
C) The odds of death, myocardial infarction, ischemia-driven revascularization, or stent thrombosis at 48 hours after randomization in the Cangrelor arm were 22% less than in the Clopidogrel arm with the true population effect between 34% and 7%. This result was statistically significant.
Summary
This is a very basic introduction to interpreting odds ratios, confidence intervals and p values only and should help healthcare students begin to make sense of published research, which can initially be a daunting prospect. However it should be stressed that any results are only valid if the study was well designed and conducted, which highlights the importance of critical appraisal as a key feature of evidence based medicine.
I do hope you enjoyed working through this and would appreciate any feedback on the content, design and presentational aspects of this tutorial.
Self test Answers
Concept check 1. The correct answer is A.
Concept check 2. The correct answer is B.
Concept check 3. The correct answer is A.
Bringing it all together – Real world example. The correct answer is C.
You may also be interested in these blogs:
Why should students know about kappa value?
Efficacy of drugs: 3 examples to get you to truly understand Number Needed to Treat (NNT)
Key to statistical result interpretation: P-value in plain English
Surrogate endpoints: pitfalls of easier questions
How did they determine diagnostic thresholds: the stories of anemia and diabetes
References:
Bhatt DL, Stone GW, Mahaffey KW, Gibson CM, Steg PG, Hamm CW, Price MJ, Leonardi S, Gallup D, Bramucci E, Radke PW, Widimský P, Tousek F, Tauth J, Spriggs D, McLaurin BT, Angiolillo DJ, Généreux P, Liu T, Prats J, Todd M, Skerjanec S, White HD, Harrington RA. CHAMPION PHOENIX Investigators. (2013). Effect of platelet inhibition with cangrelor during PCI on ischemic events. N Engl J Med. Apr 4;368(14):1303-13.
No Comments on A beginner’s guide to interpreting odds ratios, confidence intervals and p-values
Good help . thanks
17th August 2014 at 3:33 amHi Tim
Thank you so much for your informative posts- you really explain this crazy terminology in a way that we can all understand.
Do you have any experience in working with Epi Info?
Many Thanks
14th June 2014 at 9:45 pmCeleste
Thanks Celeste,
Glad it’s been of use to you. I’ve not much experience of epidemiology, but if there was anything in particular you were stuck with let me know and perhaps if I didn’t know the answer it might be fun to find it out?
Kind regards
Tim
9th September 2014 at 12:08 pmI’m just want answer for my question.
11th June 2014 at 10:14 amIs Odds Ratio always with in the CI (in between the interval)?
Tadesse
Hi Tadesse,
Yes always, the OR is the estimate of an intervention effect in a study population, which is of course only a representative sample of the whole population.
The confidence interval is always either side of this because it represents the uncertainty around the intervention effect.
Regards
Tim
9th September 2014 at 12:13 pmplease I need to know what adjusted odds ratio,crude odds ratio,bivarate and multi varate analysis, logistic regression are.
15th May 2014 at 3:13 pmHi Shemsu,
This reply might be a bit over simplified, but hopefully it helps.
Crude Odds Ratio – the odds ratio calculated using just the odds of an outcome in the intervention arm divided by the odds of an outcome in the control arm.
Adjusted Odds Ratio – is the crude odds ratio produced by a regression model which has been modified (adjusted) to take into account other data in the model that could be for instance a prognostic baseline variable.
Bivariate – analysis with two variables
Multivariate – analysis with more than two variables (it’s more complex than this, but don’t worry unless you want to be a statistician).
Logistic regression – a type of statistical model used to evaluate a binary outcome e.g. mortality (dead or alive).
Regards
Tim
9th September 2014 at 12:44 pmhi Tim
26th April 2014 at 10:47 pmfirst i would like to thank you for this amazing work its really helped me
I’m a senior medical student and i have an assignment to do about EBM I’ve been struggling with the critical appraisal especially calculating the confidence intervals around the ARR and NNT so i used a web for the calculation but now i cannot interpret the result so please may you kindly tell me what this results mean (95% CI around ARR is -0.001 to 0.073) and ( 95%CI around NNT is -782 to 14 )
Best Regards
Amal
Thanks Amal,
This is a great question and an area I’m not so certain about, so if there are any statisticians that read this post any additional answer would be very welcome.
My suggestion on this is to first consider that the NNT is calculated as the reciprocal of the absolute risk reduction (ARR). This is easily done on a standard scientific calculator by entering the ARR and then hitting the (x-1) key then( =) . The confidence interval for the NNT is done the same way with the lower limit of the ARR 95%CI and subsequently the upper limit of the ARR 95%CI.
Its worth noting at this point that ARR is an absolute measure so the line of no difference is 0 (There is an explanation of this in a slightly different context in the reply i posted for Kates question). Essentially it means that if the upper and lower limit of the ARR 95%CI are either both positive or both negative then there is a significant difference between arms. Or if one is negative and one is positive it indicates that there is not a significant difference.
However the NNT confidence interval can produce some intriguing numbers, which at first seem quite baffling. This essentially arises from the fact that if:
NNT = the reciprocal of ARR
Then if the ARR 95%CI is from a lower limit which is a negative number to an upper limit which is a positive number it must therefore pass through 0.
So, the reciprocal of 0 is ∞ (infinity).
The next point to consider is that if the NNT indicates the number needed treat for a desirable outcome, for instance if a randomised placebo controlled trial of an antiplatelet therapy for the prevention of stroke with an outcome of mortality had an ARR 0.1 (95%CI -0.05, to 0.25).
Then NNT = 10, therefore 10 people would need to be treated to prevent 1 death.
However the reciprocal of -0.05 the lower limit of the ARR 95% CI was -20 that is for every 20 people treated there is one more death. This is of course the number needed to harm NNH.
And lastly the upper limit of the ARR 95% CI was 4.
This means that an ARR of 0.1 (95%CI -0.05, to 0.25) Gives an NNT of 10 (95%CI -20, to 4), which of course at first glance seems bizarre because it appears to exclude the point estimate of 10. However taking into account how it’s calculated it makes sense?
So a more precise way of writing this taking into account 0 would be. NNT = 10 (95% CI -20 to -∞, to 4 to ∞), which does include the point estimate of 10.
So in your example: “(95% CI around ARR is -0.001 to 0.073) and (95%CI around NNT is -782 to 14)”
The intervention point estimate is not mentioned, but the upper limit 95%CI indicates that in the true population we can be 95% certain that the benefit may be as much as NNT 14 and the lower limit 95%CI indicates that in the true population we can be 95% certain that the harm may be as little as NNH 782.
If anyone has any further comments on this i would be delighted to read them.
Many thanks
Tim
23rd May 2014 at 12:29 pmvery helpful!!! thank you
17th April 2014 at 9:41 pmHi there,
I’m trying to understand the confidence intervals of a study, and really struggling! Here is part of the study:
25th April 2014 at 5:34 amBleeding time associated with ticagrelor was slightly reduced
following administration of desmopressin. On Day 5, at 25 h postticagrelor
dose, median bleeding time with ticagrelor plus desmopressin
(75 min; range 3–17 min) was slightly reduced compared
with ticagrelor alone (105 min; range 3–25 min). However, by
24 h post-ticagrelor dose, a difference between treatments was no
longer evident. Geometric least square (GLS) mean AUEC was
1751 min.h for ticagrelor plus desmopressin vs. 2208 min.h for
ticagrelor alone, difference in GLS mean 4465 (95% CI: 9047,
117) (Fig. 1).
Inhibition of platelet aggregation by ticagrelor was not affected
by co-administration of desmopressin (Fig. 2). Following ticagrelor,
mean final-extent IPA was >87% at all measured time points
(≤24 h post-dose) in the presence and absence of desmopressin.
Mean final-extent peak IPA (IPAmax) was 9932% for ticagrelor
plus desmopressin compared with 9937% for ticagrelor alone,
difference in GLS mean 005 (95% CI = 072, 063). IPAmax
measurements were calculated for 17 of the 18 volunteers (n = 17)
as one volunteer’s plasma samples were unsuitable for IPA
determination.
Hi, thank you very much for your help. I have try to find in many pages how can I interpret A mul- tivariate analysis demonstrated that the independent risk factors for developing nosocomial B. cenocepacia bacter- emia were hospitalization at the center for long-term sup- port (OR 28.8; 95% CI 1.83–453.4) and reduced use of antibiotics during the last month (OR 0.07; 95% CI 0.01– 0.40).
Also I don’t understand why this figures are so big. I’m not good at statistics and I will really appreciate your help.
In multivariate analysis, the independent risk factors for developing nosocomial B. cenocepacia bacteremia were hospitalization at the Long Term Support Center for chil- dren (OR 27.65; 95% CI 1.66–460.69) and reduced use of antibiotics during the preceding month (OR 0.05; 95% CI 0.09–0.35).
.Significantly more episodes of severe hypotension developed during the current admission among the cases (11/17) than among controls (40/44; p = 0.013). None of the patients with B. cenocepacia bacteremia died, while 7/44 of the controls died (p = 0.08).
Thank you very much for your help
27th April 2014 at 3:50 amHi Kate,
I think this is the study:
The effect of desmopressin on bleeding time and platelet aggregation in healthy volunteers administered ticagrelor.
Available online at:
http://onlinelibrary.wiley.com/doi/10.1111/jcpt.12130/pdf
Thank you for this question, indeed the interpretation of the confidence interval is different with this study because the outcome was a continuous variable, which was time. This was calculated as the Geometric Least Square (GLS) mean, which for the purposes of this explanation we will consider to be simply a mean and ignore the GLS part.
So as outlined in the tutorial above an odds ratio is a relative measure of effect so the line of no difference is one. However a mean is an absolute measure of effect so the line of no difference is 0.
For example if a placebo controlled randomised controlled trial evaluated the effect of a new medication to reduce blood pressure, the results might show:
Intervention arm mean reduction in BP 10mmHg
Placebo arm mean reduction in BP 2mmHg
So the intervention arm has a reduced mean BP of -8mmHg compared to the placebo arm.
It’s worth noting at this point that if both had reduced BP by 10mmHg then the difference in mean reduction in BP between arms would be 0. That is there was no difference, hence the line of no difference in absolute measures of effect is 0.
Having now established the point estimate we can think about the confidence interval.
Therefore if:
The intervention arm has a reduced mean BP by -8mmHg compared to the placebo arm and the confidence interval is (95%CI: -12mmHg, to -4mmHg). Then this would indicate that the new blood pressure medication reduces mean blood pressure by 8mmHg in the population in the study and we can be 95% certain that the true population reduction is from 12mmHg lower to 4mmHg lower and because the confidence interval does not cross 0 it is statistically significant.
However if:
The intervention arm has a reduced mean BP by -2mmHg compared to the placebo arm and the confidence interval is (95%CI: -8mmHg, to 4mmHg). Then this would indicate that the new blood pressure medication reduces mean blood pressure by 2mmHg in the population in the study and we can be 95% certain that the true population reduction is from 8mmHg lower to 4mmHg higher and because the confidence interval does cross 0 it is not statistically significant.
I hope this example makes sense?
Back to your example:
“24 h post-ticagrelor dose, a difference between treatments was no longer evident. Geometric least square (GLS) mean AUEC was 175.1 min.h for ticagrelor plus desmopressin vs. 220.8 min.h for ticagrelor alone, difference in GLS mean -44.65 (95% CI: -90.47, 1.17)”
Therefore although GLS mean AUEC was lower in the ticagrelor plus desmopressin arm compared to ticagrelor alone arm the confidence interval is from a minus number -90.47 to a positive number 1.17 passing through the line of no difference 0 suggesting that there is no significant difference between arms for the outcome of bleeding time at the 24 hrs post ticagrelor dose.
The second example in your question can be interpreted in the same way.
Regards
Tim
21st May 2014 at 12:14 pmHi Kate,
I think this is the study:
The effect of desmopressin on bleeding time and platelet aggregation in healthy volunteers administered ticagrelor.
Available online at:
http://onlinelibrary.wiley.com/doi/10.1111/jcpt.12130/pdf
Thank you for this question, indeed the interpretation of the confidence interval is different with this study because the outcome was a continuous variable, which was time. This was calculated as the Geometric Least Square (GLS) mean, which for the purposes of this explanation we will consider to be simply a mean and ignore the GLS part.
So as outlined in the tutorial above an odds ratio is a relative measure of effect so the line of no difference is one. However a mean is an absolute measure of effect so the line of no difference is 0.
For example if a placebo controlled randomised controlled trial evaluated the effect of a new medication to reduce blood pressure, the results might show:
Intervention arm mean reduction in BP 10mmHg
Placebo arm mean reduction in BP 2mmHg
So the intervention arm has a reduced mean BP of -8mmHg compared to the placebo arm.
It’s worth noting at this point that if both had reduced BP by 10mmHg then the difference in mean reduction in BP between arms would be 0. That is there was no difference, hence the line of no difference in absolute measures of effect is 0.
Having now established the point estimate we can think about the confidence interval.
Therefore if:
The intervention arm has a reduced mean BP by -8mmHg compared to the placebo arm and the confidence interval is (95%CI: -12mmHg, to -4mmHg). Then this would indicate that the new blood pressure medication reduces mean blood pressure by 8mmHg in the population in the study and we can be 95% certain that the true population reduction is from 12mmHg lower to 4mmHg lower and because the confidence interval does not cross 0 it is statistically significant.
However if:
The intervention arm has a reduced mean BP by -2mmHg compared to the placebo arm and the confidence interval is (95%CI: -8mmHg, to 4mmHg). Then this would indicate that the new blood pressure medication reduces mean blood pressure by 2mmHg in the population in the study and we can be 95% certain that the true population reduction is from 8mmHg lower to 4mmHg higher and because the confidence interval does cross 0 it is not statistically significant.
I hope this example makes sense?
Back to your example:
“24 h post-ticagrelor dose, a difference between treatments was no longer evident. Geometric least square (GLS) mean AUEC was 175.1 min.h for ticagrelor plus desmopressin vs. 220.8 min.h for ticagrelor alone, difference in GLS mean -44.65 (95% CI: -90.47, 1.17)”
Therefore although GLS mean AUEC was lower in the ticagrelor plus desmopressin arm compared to ticagrelor alone arm the confidence interval is from a minus number -90.47 to a positive number 1.17 passing through the line of no difference 0 suggesting that there is no significant difference between arms for the outcome of bleeding time at the 24 hrs post ticagrelor dose.
The second example in your question can be interpreted in the same way.
Regards
Tim
21st May 2014 at 12:19 pmThanks for your example above. It helps a lot. Why do we take the odds ratio and -1 in order to get the %. Eg 0.78 would mean 22% less and 1.78 would mean 22% greater…
5th April 2014 at 1:39 amAll comments have been very helpful thanks
Hello Andrea,
The odds ratio is calculated by dividing the odds in the intervention arm by the odds in the control arm.
So if:
Odds in the intervention arm = 5
Odds in the control arm = 10
Then the odds in the intervention arm are 50% less than in the control arm.
So the OR is 0.5 therefore the outcome in the intervention arm is 50% less than the control arm.
Conversely if:
Odds in the intervention arm = 10
Odds in the control arm = 5
Then the odds in the intervention arm are 100% greater than in the control arm.
So the OR is 2 therefore the outcome in the intervention arm is 100% more than the control arm.
So in your example of OR 0.78 it would be 22% less and the example of 1.78 it would be 78% greater.
Remember though the importance of confidence intervals and interpreting data in the context of the totality of evidence.
Kind regards
Tim
14th April 2014 at 8:14 pmHi Tim, I am studying for a health technology exam… need to learn the formula for calculating a confidence interval but there seems to be a number of different formulas around and am a little confused. Any suggestions? Thanks
17th April 2014 at 1:03 pmHi Sally,
Will you have to calculate the CI for an OR? Can you let me know what sort of thing you have an interest in and I will post a relevant worked example for you.
Tim
22nd April 2014 at 10:15 pmI would like to ask how to interpret CI like (1;1,3) ?
TIA
25th March 2014 at 6:35 pmHello,
Could you possibly give me a bit more detail and send me a link to where it comes from so I can see it in context.
Thanks
Tim
27th March 2014 at 1:16 pmTim, thanks for the great, concise tutorial. You have filled in the gaps where my texts left holes in my understanding. Greatly appreciated.
21st March 2014 at 7:25 pmThe sentence in my paper was :
The most active quartile of women had a similar risk of breast cancer as the least active (odds ratio [OR], 1.04; 95% confidence interval (CI), 0.73-–1.48). Additional adjust ment for randomized treatment assignment, body mass index, alcohol consumption, menstrual and reproductive characteristics, use of oral contraceptives and postmenopausal hormones, and family history of breast cancer did not materially change the find ings (OR, 1.10; 95% CI, 0.73–1.67)’
The way I interpreted this was :
This means the odds of having breast cancer among physically active women is 1.04 times higher/ 4% more than odds of breast cancer in females who are least active which indicates no relationship between decreased rates of breast cancer and physically active women. Because the study used a sample, we don’t know the true value is for the whole population but the best estimate is 1.04 and they are 95% confident that the true value lies within the range of 0.73 to 1.48. As the range of value includes 1 (equal odds) we can say that we don’t have statistically significant evidence that there is a bigger risk of cancer among least physically active women. Furthermore, 1.04 is close to 1 meaning the outcome is the similar in both groups, which implies there is no difference between the two arms of the study.
After adjusting for confounding factors, the odds ratio of physical activity is increased a little from 1.04 to 1.10. This result suggests that these variables were slightly strong confounders of the association between physical activity and breast cancer. But even after adjusting, the 95% confidence intervals still included 1 meaning the results weren’t statistically significant . However, Adjusting for the confounding factors gives us an odds ratio further from unity (1) which means that the protection afforded by physical activity, if any, is stronger than the initial result led us to think.
I was unsure whether I had interpreted this correctly.
16th March 2014 at 4:47 pmexcellent
19th March 2014 at 3:19 pmHi,
I’m trying to relate something I read in an article, to the way you have described it in your article.
…”an estimated odd ratio for response for anastrozole 1mg versus megestrol acetate was 1.95 (97.7% CI, 0.65-5.91)…”
Please may you kindly tell me what this means in plain english?
Thank you.
5th March 2014 at 2:14 pmHello Rupy,
I think your quote comes from the study:
A Phase III trial comparing anastrozole (1 and 10 milligrams), a potent and selective aromatase inhibitor, with megestrol acetate in postmenopausal women with advanced breast carcinoma.
Available online at:
http://onlinelibrary.wiley.com/doi/10.1002/(SICI)1097-0142(19970215)79:4%3C730::AID-CNCR10%3E3.0.CO;2-0/full
I think the plainest way to put it would be that there is no difference between anastrozole 1mg versus megestrol acetate for the outcome of “response”, which appears to be a combination of what the authors of the paper refer to as “Complete response” and “Partial response” of table 3.
Interestingly it’s a parallel three arm study looking at two different doses of double blind anastrozole and open label megestrol acetate. The total sample size is quite small at 386 participants, which perhaps goes some way to explaining the wide confidence interval (0.65-5.91), which are either side of 1 hence indicating there is not a significant difference. It is also a 97.5% confidence interval, which will also be wider than a 95% confidence interval, which I calculated (very roughly) as 0.75-5.07.
It’s also worth just keeping in mind that this is quite an old study with many of its references dating to the early to mid 90’s. So my first thought in considering the value of these drugs would be that no study stands in isolation and given that it’s quite old and has wide confidence intervals, it would be a good idea to look for a meta-analysis. Ideally start by looking for a Cochrane review and you may well find this study included in a meta-analysis, which could help with a more definitive answer to whether anastrozole or megestrol acetate is more effective for the type of patients you are interested in or perhaps they might be just as effective, but one may have more tolerable side effect or safety better profile?
So this might be of interest to you:
http://onlinelibrary.wiley.com/doi/10.1002/14651858.CD003370.pub3/abstract
I do hope this answer is of some help.
Thank you for your question, I quite enjoyed looking up this paper.
Tim
P.S. I’m conscious of the fact that this website is open to everyone not just healthcare students, so I would have to suggest that this shouldn’t be considered as medical advice and this topic is best discussed with a specialist in this field.
6th March 2014 at 12:41 amHi Tim,
Thank you for your reply, it was very helpful.
I just have a question regarding hazard ratios. Are these similar to odds ratio?
For instance, a disease free survival was longer for an anastrozole group compared to a tamoxifen group; and the statistic given was “hazard ratio 0.83 (95% CI 0.71-0.96, p value=0.013)
from this statistic I understand it is statistically significant, with a p value below 0.05. But would you be able to kindly explain what this hazard ratio means?
Thank you.
Rupy
16th March 2014 at 6:13 pmHello Rupy,
Thanks for the feedback and I’m glad it was some use to you.
The interpretation of a hazard ratio is essentially the same as an odds ratio.
However it’s probably worth noting that whilst an odds ratio is derived from calculating the odds of an event in the intervention and the control arms expressed as a ratio.
The hazard ratio is derived from calculating the rate (number of events/time) in the intervention and the control arms expressed as a ratio. So there are some additional statistical considerations, but that would perhaps be more detail than is needed for basic interpretation.
Kind regards
Tim
24th March 2014 at 4:57 pmplz tell me how to calculate CI
14th May 2015 at 2:18 pmthanx .got some idea.
14th May 2015 at 2:03 pmExcellent info. I’ve always been a little fuzzy on CI and crossing of *1*… etc. That was extremely helpful. Thanks!
5th March 2014 at 12:03 pmVery pleased it’s been of some use. Please feel free to recommend the S4BE website as widely as possible and have a look at the many great articles and blogs in the site.
Regards
Tim
6th March 2014 at 12:48 amI think it is an excellent idea to explain what statistical analysis actually means in a simplified manner this may be a little too simplified. Your explanation of odds ratio is just fine however it is important to look at how and if the researchers normalized the data. If there was no normalization for things like other health issues, differences in how skilled the surgical teams were, or the relative “smoothness” of the procedure then it is possible that these or other factors are responsible for some or all of the observed differences in outcomes. A confidence interval is actually a probabilistic statement about the repeatability of the trial as a whole (with a different set of patients who meet the same criteria) so saying that the confidence interval is 0.4-0.6 at a 95% level is actually saying there is if they performed this trial over and over they estimate that 95% of the trials will produce a result that is between .4 and .6. I know it sounds like I just said what you did but in a more complicated way but the way answer B is phrased discounts the 5% chance that the trial is actually an outlier which would result in misleading conclusions being drawn from its result. As far as P Value goes you are correct that a P Value of .05 is frequently stated and it is indeed often the threshold for statistically significant; however “statistically significant;” is closer to a legal term than a statement of fact. A P-Value of .05 simply means that assuming the individual data points are Normally distributed (which they almost always are) then there is at most a 5% chance that there is no correlation between the manipulated variables and the results observed. I don’t know if this will help or just confuse people all over again because statistics consistently cause smart people to have a logical but incorrect understanding of what a set of statistics mean in a practical sense.
18th January 2014 at 3:16 pmThanks very much Ben,
This comment is very much appreciated and I’m sure will help visitors to the S4BE website.
Regards
Tim
20th January 2014 at 6:46 pm