A beginner’s guide to interpreting odds ratios, confidence intervals and p-values
Posted on 13th August 2013 by Tim Hicks
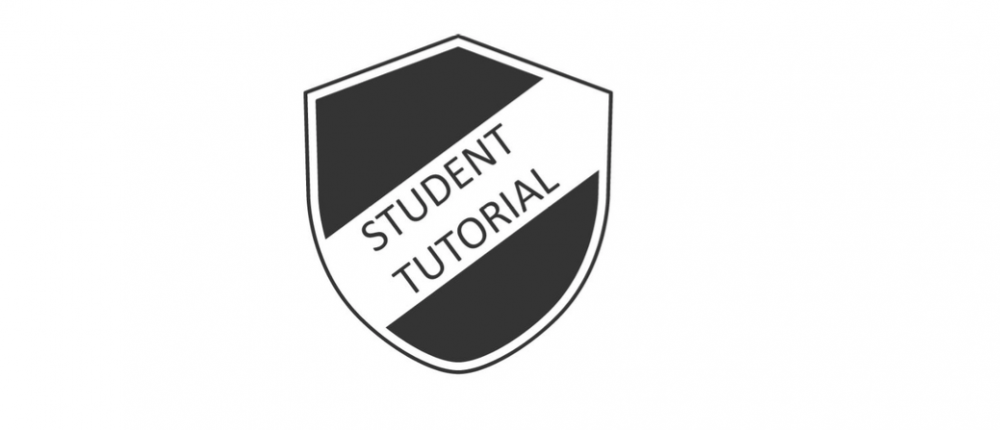
Who is it for?
Students of medicine or from the clinical sciences and professions allied to medicine wanting to enhance their understanding of medical literature they will encounter throughout their careers.
What will I learn and how?
How to interpret odds ratios, confidence intervals and p values with a stepwise progressive approach and a’concept check’ question as each new element is introduced.
How long will it take?
Approximately 20 minutes.
What it is not
A statistical textbook reworded or how to calculate any of these statistics.
Contents:
Introduction
Odds ratio
Confidence interval
P value
Bringing it all together – Real world example
Summary
Self test Answers
Introduction
The first steps in learning to understand and appreciate evidence-based medicine are daunting to say the least, especially when confronted with the myriad of statistics in any paper. This short tutorial aims to introduce healthcare students to the interpretation of some of the most commonly used statistics for reporting the results of medical research.
The scenario for this tutorial is centred around the diagram below, which outlines a fictional parallel two arm randomised controlled trial of a new cholesterol lowering medication against a placebo.
Odds ratio (OR)
An odds ratio is a relative measure of effect, which allows the comparison of the intervention group of a study relative to the comparison or placebo group.
So when researchers calculate an odds ratio they do it like this:
The numerator is the odds in the intervention arm
The denominator is the odds in the control or placebo arm = Odds Ratio (OR)
So if the outcome is the same in both groups the ratio will be 1, which implies there is no difference between the two arms of the study.
However:
If the OR is > 1 the control is better than the intervention.
If the OR is < 1 the intervention is better than the control.
Concept check 1
If the trial comparing SuperStatin to placebo with the outcome of all cause mortality found the following:
Odds of all cause mortality for SuperStatin were 0.4
Odds of all cause mortality for placebo were 0.8
Odds ratio would equal 0.5
So if the trial comparing SuperStatin to placebo stated OR 0.5
What would it mean?
A) The odds of death in the SuperStatin arm are 50% less than in the placebo arm.
B) There is no difference between groups
C) The odds of death in the placebo arm are 50% less than in the SuperStatin arm.
Confidence interval (CI)
The confidence interval indicates the level of uncertainty around the measure of effect (precision of the effect estimate) which in this case is expressed as an OR. Confidence intervals are used because a study recruits only a small sample of the overall population so by having an upper and lower confidence limit we can infer that the true population effect lies between these two points. Most studies report the 95% confidence interval (95%CI).
If the confidence interval crosses 1 (e.g. 95%CI 0.9-1.1) this implies there is no difference between arms of the study.
Concept check 2
So if the trial comparing SuperStatin to placebo stated OR 0.5 95%CI 0.4-0.6
What would it mean?
A) The odds of death in the SuperStatin arm are 50% less than in the placebo arm with the true population effect between 20% and 80%.
B) The odds of death in the SuperStatin arm are 50% less than in the placebo arm with the true population effect between 60% and 40%.
C) The odds of death in the SuperStatin arm are 50% less than in the placebo arm with the true population effect between 60% and up to 10% worse.
P values
P < 0.05 indicates a statistically significant difference between groups. P>0.05 indicates there is not a statistically significant difference between groups.
Concept check 3
So if the trial comparing SuperStatin to placebo stated OR 0.5 95%CI 0.4-0.6 p<0.01
What would it mean?
A) The odds of death in the SuperStatin arm are 50% less than in the placebo arm with the true population effect between 60% and 40%. This result was statistically significant.
B) The odds of death in the SuperStatin arm are 50% less than in the placebo arm with the true population effect between 60% and 40%. This result was not statistically significant.
C) The odds of death in the SuperStatin arm are 50% less than in the placebo arm with the true population effect between 60% and 40%. This result was equivocal.
Bringing it all together – Real world example
A drug company-funded double blind randomised controlled trial evaluated the efficacy of an adenosine receptor antagonist Cangrelor vs Clopidogrel in patients undergoing urgent or elective Percutaneous Coronary Intervention (PCI) who were followed up for specific complications for 48 hrs as outlined in the diagram below (Bhatt et al. 2009).
The results section reported “The rate of the primary efficacy end point was … (adjusted odds ratio with Cangrelor, 0.78; 95% confidence interval [CI], 0.66 to 0.93; P=0.005)
What does this mean?
A) The odds of death, myocardial infarction, ischemia-driven revascularization, or stent thrombosis at 48 hours after randomization in the Cangrelor arm were 22% less than in the Clopidogrel arm with the true population effect between 34% and 7%. This result was not statistically significant.
B) The odds of death, myocardial infarction, ischemia-driven revascularization, or stent thrombosis at 48 hours after randomization in the Cangrelor arm were 34% less than in the Clopidogrel arm with the true population effect between 7% and 22%. This result was statistically significant.
C) The odds of death, myocardial infarction, ischemia-driven revascularization, or stent thrombosis at 48 hours after randomization in the Cangrelor arm were 22% less than in the Clopidogrel arm with the true population effect between 34% and 7%. This result was statistically significant.
Summary
This is a very basic introduction to interpreting odds ratios, confidence intervals and p values only and should help healthcare students begin to make sense of published research, which can initially be a daunting prospect. However it should be stressed that any results are only valid if the study was well designed and conducted, which highlights the importance of critical appraisal as a key feature of evidence based medicine.
I do hope you enjoyed working through this and would appreciate any feedback on the content, design and presentational aspects of this tutorial.
Self test Answers
Concept check 1. The correct answer is A.
Concept check 2. The correct answer is B.
Concept check 3. The correct answer is A.
Bringing it all together – Real world example. The correct answer is C.
You may also be interested in these blogs:
Why should students know about kappa value?
Efficacy of drugs: 3 examples to get you to truly understand Number Needed to Treat (NNT)
Key to statistical result interpretation: P-value in plain English
Surrogate endpoints: pitfalls of easier questions
How did they determine diagnostic thresholds: the stories of anemia and diabetes
References:
Bhatt DL, Stone GW, Mahaffey KW, Gibson CM, Steg PG, Hamm CW, Price MJ, Leonardi S, Gallup D, Bramucci E, Radke PW, Widimský P, Tousek F, Tauth J, Spriggs D, McLaurin BT, Angiolillo DJ, Généreux P, Liu T, Prats J, Todd M, Skerjanec S, White HD, Harrington RA. CHAMPION PHOENIX Investigators. (2013). Effect of platelet inhibition with cangrelor during PCI on ischemic events. N Engl J Med. Apr 4;368(14):1303-13.
No Comments on A beginner’s guide to interpreting odds ratios, confidence intervals and p-values
Very cogent explanation. Thanks. Kavitha
15th November 2018 at 9:37 amIt would have been good if the etymology of terms were added.
12th September 2018 at 12:23 pmwhat does 95% mean ? Is it the measure of confidence meaning ;that we are 95% confident of the applicable range’s(between 40% and 60%) dependability for its application for general population?
very informative
29th August 2018 at 7:44 pmNeeded clear, concise help in a hurry and you provided it. Thank you!
20th August 2018 at 2:26 amThank you ! This helped me understand aspect of biostats better than any book.
23rd July 2018 at 6:50 pmHi, I’m a little confused about the CI interval and your statement that even if it’s 95% if the interval “crosses” 1 it’s not statistically significant. I am reading a paper comparing the effects of declawing of cats on various adverse out comes as compared with non declawed cats. In all of the comparisons (OR were calculated) the CI was 95% but the values listed under the CI were often greater than 1. Examples include the following (1.99-7.84, 1.32 – 4.56)
14th February 2018 at 3:47 pmHi Tim
I am running a Vaccine effectiveness study and wanted to calculate the Vaccine effectiveness from OR. The OR is 27 (CI 17-40). This translates to VE of 73 (calculated as 1-OR). Do the confidence intervals also get changed to 60-83?
21st December 2017 at 5:44 pmThanks for your response.
I read this article completely concerning the resemblance of most up-to-date and earlier
5th December 2017 at 5:09 pmtechnologies, it’s awesome article.
There is some nice introductory stuff here but I’m concerned that your explanation of p values feeds into popular misconceptions that are somewhat harmful (see comment 91 for a stark example).
The p value is the risk of obtaining the observed result, or a more extreme result, by chance if the null hypothesis were true. 0.05 was chosen by a researcher many decades ago and the entire biomedical science community followed suit. There is nothing magical about 0.05 that makes a result “significant”.
I think it’s much more important that students learn about the scientific method, hypothesis testing, trial design, data types and distribution etc. before worrying about significance testing. Otherwise the risk is that they go through their entire career mistakenly looking for the magical 0.05 like comment no.91
26th November 2017 at 7:52 pmHi Tim,
30th September 2017 at 8:37 amFelt I should just say I thought this little intro to OR and CI was excellent.
I’m a 67-year-old (full-time) neurosurgeon down south and for years, I’ve always gone by p levels in assessing stats presented in papers. Never worried much about the niceties of CI. I read your very clear article and realise now I should have done this years ago! Well done and thanks.
Hi,
Can somebody please help me? Can somebody explain this (AOR= 0,04 95% CI 0,02-0,09)? It would mean that an anterior and an lateral posterior episiotomie would be the best intervention to prevent an intrapartumhemmorage. Kindly help.
3rd September 2017 at 4:00 pmHi Tim,
I am a current post-graduate fellow working with the LA County Department of Public Health. While wrapping up my epidemiological research project I searched for a quick refresher or reference guide for this very topic. You awesome data came up. Thank you so very much for compiling this information in a quick and straight forward manner. It helped me expedite my review of ORs essential to completing the data analysis portion of my manuscript.
You’re so awesome!!!
21st March 2017 at 9:31 pmLet us consider the relationship between smoking and lung cancer. Suppose exposure to cigarette smoke increases the incidence of lung cancer by 20% (i.e. the relative risk is 1.2). Lung cancer has a baseline incidence of 3% per year (in the non-exposed group). Suppose as well that baseline incidence in obese individuals is 1/3 less (i.e. 1%/yr.), and the relative risk associated with the exposure is 1.2. You follow up 1000 non-obese and 1000 obese subjects with the exposure, and an equivalent number without the exposure. The study lasts 25 years. Work with 25-year cumulative incidence and a denominator of 1000.
How to calculate this problem? Especially the construction of the table. Kindly help.
18th February 2017 at 3:59 amDo you mind if I quote a feww of your articles as long as I provide credit and
5th February 2017 at 5:59 amsources back to your webpage? My blog site is in the very same
niche as yours and my visitors would certainly benefit from some of
the information you proviude here. Pleae let mee know if this alright with you.
Thanks!
You’re welcome to quote any of the articles if you provide credit and link back to the original source. Many thanks!
6th February 2017 at 9:28 amfantastic resource, thanks so much!!
29th November 2016 at 4:08 amMight you help me understand the following interpretation?:
“Group A reported significantly less difficulty in the instrumental activities of daily living (IADL) than the control group (effect size, 0.29; 99% confidence interval [CI], 0.03-0.55). Neither Group B (effect size, 0.26; 99% CI, −0.002 to 0.51) nor Group C (effect size, 0.20; 99% CI, −0.06 to 0.46) had a significant effect on IADL”
What is the basis for interpreting no significant effect in groups B and C?
2nd October 2016 at 7:09 pmHi Tim, your explanation is so much easy to understand. Just a question. Is Odds ratio the same as relative risk ratio?
30th September 2016 at 5:55 amAlso I have difficulty understanding different study designs and ends up misinterpreting them.
Is there an easier way of understanding the difference between cohort studies, case control studys, retrospective cohort studies and cross-sectional studies
When doing a lit review, I find that results are frequently presented in different ways. I’d like to be able to convert them to read the same way so that I can compare them.
I know that I can convert OR1 by using the equation 1/x, where x=OR<1, which then reverses the factors being compared. For example,
FB is less likely in rural [OR=.26 (.12, .50)] than urban areas
converts to:
FB is more likely in urban [OR=3.85 (2.00, 8.33] vs rural areas
My question is how to convert:
HB is more likely in rural [OR=22.8 (10.6, 49.4)] than urban areas
Is it correct to say:
FB is more likely in urban [OR=22.8 (10.6, 49.4)] than rural areas
Or is there some calculation that needs to be done? FB and HB are dichotomous outcomes.
Thank you.
20th September 2016 at 7:17 pmOdds Ratio (OR) is a measure associations between exposure (risk factors) and the incidence of disease; calculated from the incidence of the disease in at risk groups (exposed to risk factors) compared to the incidence of the disease in non-risk group (not exposed to a risk factor).
In this present study, by cross sectional study, We got OR 2.8 for variable keep livestock such as goats, sheep and pigs. Is this meaning Respondents or household who keep livestock such as goats, sheep and pigs have a 2.8 times greater chance of contracting malaria compared to a respondents or household who do not raise cattle where the confidence interval [CI: 2.180 – 3492])?
What does different between OR and RR, for this case
Regards for your advice
22nd July 2016 at 9:12 ami know how to calculate crude odds ratio manually but how can i calculate adjusted odds ratio manually ?
10th June 2016 at 9:49 am