Statistical significance vs. clinical significance
Posted on 23rd March 2017 by Cindy Denisse Leyva De Los Rios
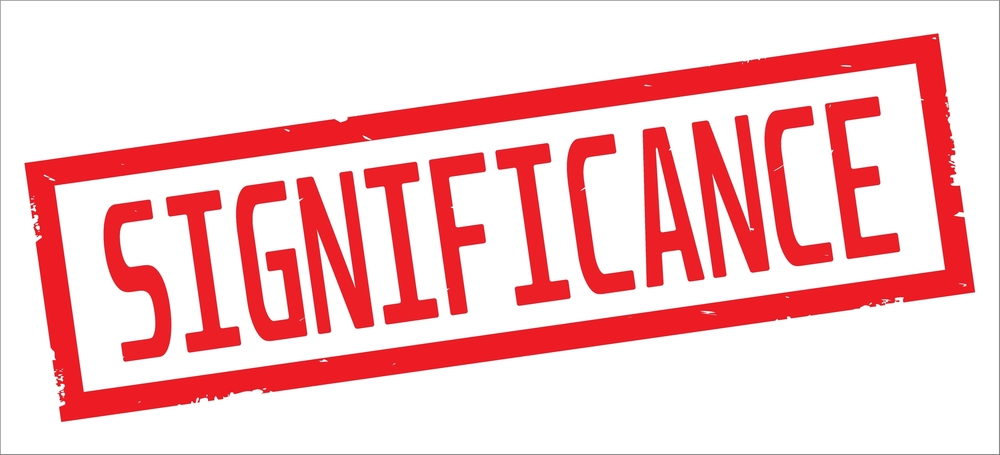
What if I told you that I conducted a study which shows that a single pill can significantly reduce tiredness without any adverse effects?
Would you try it? Or recommend it? Or would you want more information to decide? Maybe you’re a little skeptical. I will show you the results, so don’t make a decision just yet.
From now on let’s imagine this scenario…
Before I tell you the results of my study, you need to know how it was carried out.
- First I took a group of 2,000 adults between 20-30 years old, all of whom suffer from constant tiredness. Then the participants were randomly divided into 2 groups, with 1000 participants in each.
- One group of participants (the intervention group) were given the new drug: energylina. The other groups of participants (the control group) were given a dummy (placebo) pill.
- Nobody knew – neither the participants nor the researchers involved in the experiment – whether they were taking ‘energylina’ or the placebo. The participants took the pills for 3 weeks, 2 per day.
- We used a scale to measure participants’ levels of tiredness before and after the trial. This rated fatigue on a scale of 1 to 20; with 1 meaning the participant felt entirely well-rested and 20 meaning the participant felt entirely fatigued.
- The results revealed that:Â 90% of the participants in the energylina group improved by 2 points on the scale. 80% of participants in the placebo group improved by 1 point on the scale.
- This difference between the groups was statistically significant (p < 0.05) meaning that, at the end of the 3 weeks, participants in the intervention group were significantly less tired than those in the control group.
So does that mean the treatment is effective? Should you take “energylina”? Should every doctor prescribe it?
Not necessarily! Let’s make a couple of things clear first. At this point, you might be wondering why the title of this blog ‘statistical significance vs. clinical significance’.
Well, I will explain it right now; the results I gave you are there to help you make a decision. You want to know whether energylina is effective enough to recommend to individuals who suffer fatigue. Did the results convince you?
Before you answer, first let me clarify something: Clinical significance is the practical importance of the treatment effect, whether it has a real, palpable, noticeable effect on daily life. For example, imagine a safe treatment that could reduce the number of hours you suffered with flu-like symptoms from 72 hours to 10 hours. Would you buy it? Yes, probably! When we catch a cold, we want to feel better, as quickly as possible. So, in simple terms, if a treatment makes a positive and noticeable improvement to a patient, we can call this ‘clinically significant’ (or clinically important).
In contrast, statistical significance is ruled by the p-value (and confidence intervals). When we find a difference where p <0.05, we call this ‘statistically significant’. Just like our results from the above hypothetical trial. If a difference is statistically significant, it simply means it was unlikely to have occurred by chance. It doesn’t necessarily tell us about the importance of this difference or how meaningful it is for patients.
So it’s important to consider that trial results could be…
- Statistically significant AND clinically important. This is where there is an important, meaningful difference between the groups and the statistics also support this. (The flip side of this is where a difference is neither clinically nor statistically significant).
- Not statistically significant BUT clinically important. This is most likely to occur if your study is underpowered and you do not have a large enough sample size to detect a difference between groups. In this case you might fail to detect an important difference between groups.
- Statistically significant BUT NOT clinically important. This is more likely to happen the larger sample size you have. If you have enough participants, even the smallest, trivial differences between groups can become statistically significant. It’s important to remember that, just because a treatment is statistically significantly better than an alternative treatment, does not necessarily mean that these differences are clinically important or meaningful to patients.
Going back to our hypothetical study, what have we got: statistical significance? clinical significance, or both?
Remember we had 2 groups, with 1000 participants in each. In the intervention group, 90% of the participants improved by 2 points on the tiredness scale whereas 80% of the participants in the placebo group improved by 1 point on the tiredness scale.
Is the difference between both groups remarkable? Would you buy my product to have a slightly higher probability of achieving 1 point less on a tiredness scale, compared with taking nothing? Perhaps not. You might only be willing to take this new pill if it were to lead to a bigger, more noticeable benefit for you. For such a small improvement, it might not be worth the cost of the pill. So although the results may be statistically significant, they may not be clinically important.
To avoid falling in the trap of thinking that because a result is statistically significant it must also be clinically important, you can look out for a few things…
- Look to see if the authors have specifically mentioned whether the differences they have observed are clinically important or not.
- Take into account sample size: be particularly aware that with very large sample sizes even small, unimportant differences may become statistically significant.
- Take into account effect size. In general, the larger the effect size you have, the more likely it is that difference will be meaningful to patients.
So to conclude, just because a treatment has been shown to lead to statistically significant improvements in symptoms does not necessarily mean that these improvements will be clinically significant (i.e. meaningful or relevant to patients). That’s for patients and clinicians to decide.
No Comments on Statistical significance vs. clinical significance
I am really impressed how accurately you explained such a difficult & crucial topic. Being a 3rd year medical student & to write this blog in a well constructed evidence-based manner is overwhelming..I wish you all the best hoping one day you can be able to join the Cochrane group & conduct clinical trials that will make a difference in the medical field.
24th February 2018 at 4:10 pmEvidence-based medicine is the new god. Nothing replaces common sense and logic … or does it? I agree with what is clinically relevant, it makes sense. Thank you!
1st February 2018 at 2:04 pmWell said!
17th January 2018 at 7:18 amThis will really help in decision making.
A good article. Also great comments. The issue of statistical significance and clinical significance has generated a lot constructive arguments at different level of biomedical researches, as we can also see here. But the fact is that statistical significance cannot be wholly accepted as clinical significance. You can agree with me that statistical significance is a necessary condition but not a sufficient condition for clinical significance.
17th October 2017 at 5:48 pmI am not agree with all aurguments presented here. What I know is that increase in sample size doesn’t provide significance if there is no effect. Whenever, statistical significance and clinical or scientific significance are not equivalent then you need to assess your study or experimental settings for scientific validations again.
6th June 2017 at 4:18 pmYou need to know the concept “asymptotic”.the concept belongs to derivatives, a rate of change problem and useful to understand increase in sample size and significance. Increasing sample size can not convert non-significance into significance.
And what you said in you article like cost and other thing that are not included in your study or experiment. At what cost costomers might buy the drug need statistical study but it will be more a business problem.
Thank you! I’m a Consultant Surgeon and ALWAYS find stats a challenge. this simple explanation really helps
26th May 2017 at 5:18 pmThis is a good article that clearly describes and illustrates an important point through the use of a hypothetical, yet typical, example. In clinical trials it is very common to find differences in outcomes between interventions that reach statistical significance, yet are of small magnitude. The article raises, but does not elaborate upon, another long-recognised issue that is very important and related. Clinical trials tend to illustrate the relative performance of interventions in populations, not individuals. The data are usually presented as mean values with an index of variability (SD, SE, CV) for end-of-trial absolute or between-treatment differences in predefined endpoints. But most readers (myself included) do not intuitively take account of the spread of the data and instead tend to perceive the relative ‘effects’ of the interventions tested in terms of the mean data. We mistakenly perceive the mean effect as the effect.
2nd May 2017 at 11:03 amIt is therefore possible that small-but-significant differences in the overall mean values disguise much larger clinically valuable effects in limited subgroups. Taking the article’s case history, for example, it is possible that the small relative improvement in alertness score in the overall study that was observed with Energylina versus placebo was entirely due to an improvement of much greater magnitude (and clinical relevance) in a small subgroup of individuals who had a high baseline level of tiredness. The chances are the original study would not be powered to show a statistically significant difference for this subgroup, but post hoc subgroup analyses could nevertheless inform the direction to take with future studies.
In terms of marketer of the intervention, this possibility poses a dilemma: The identification of the subgroup(s) in which the intervention is really advantageous effectively niches the product. Does the marketeer prefer a narrative that describes a small benefit for the many, or a large benefit for the few? Clearly, prescribers, regulators, payers and patients will ultimately benefit from tailored intervention informed by subgroup analysis.
Significantly explained the significant difference of trial result. ?
30th April 2017 at 4:05 pmThat’s a well explained article especially to understand the reason why drugs fail to achieve desired end point during clinical trials
29th April 2017 at 5:16 pmreally nicely explained, thanks
25th April 2017 at 5:35 amVery well explained. This is the same topic on which i am working. we all get carried away by the p value least realizing the importance of clinical or practical significance.
23rd April 2017 at 1:33 pmOutstanding!
4th April 2017 at 7:00 pmWell articulated.
28th March 2017 at 6:07 pmNicely explained, good piece of work.
26th March 2017 at 9:44 amVery well explained
25th March 2017 at 6:52 amNice article. Just one note, ‘statistically significant’ doesn’t mean that the result is unlikely to have occurred by chance. The ASA have written a nice article on interpreting p-values (http://amstat.tandfonline.com/doi/full/10.1080/00031305.2016.1154108).
24th March 2017 at 5:50 pmGreat article to read. Statisticians trying to solve the misinterpretation of statistical significance by a consensus.Amazing thing!
29th April 2017 at 5:23 pmMuy bien.
24th March 2017 at 1:41 amGood luck for your career! This article is very important for medical choises.
23rd March 2017 at 8:48 pmExcelente ¡¡¡¡¡
23rd March 2017 at 2:55 pm