Don’t confuse “statistical significance” with “importance”
Posted on 1st May 2018 by Jessica Rohmann
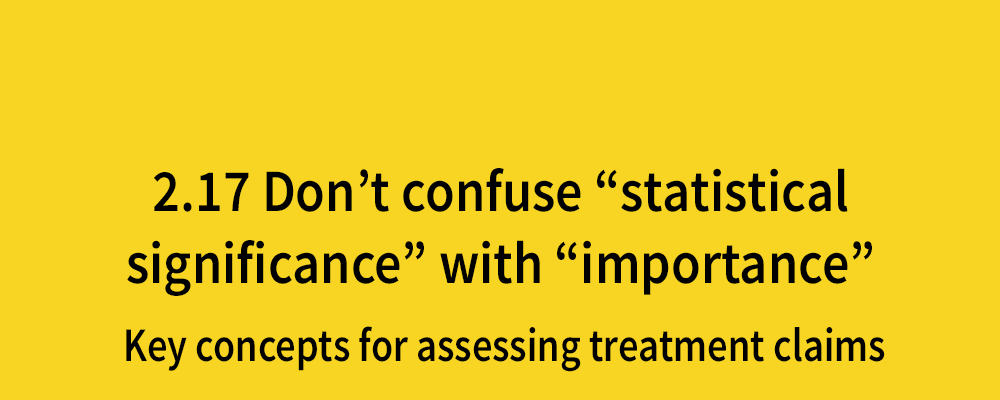
This is the twenty-ninth blog in a series of 36 blogs based on a list of ‘Key Concepts’ developed by an Informed Health Choices project team. Each blog will explain one Key Concept that we need to understand to be able to assess treatment claims.
When we read the word significant, flashing lights and sirens go off in our minds. We should care about this, we think. Synonyms such as ‘important’, ‘meaningful’, and ‘powerful’ quickly come to mind. Unfortunately, this otherwise innocent looking word has caused a significant amount of trouble in scientific circles, as its use (and abuse) in the context of interpreting results has forever coupled the word with the concept of statistical hypothesis testing (think p-values and see the previous Key Concept blog: ‘Confidence Intervals should be reported‘).
Let’s break it down…
As we discussed in the previous key concept blog, arbitrary cut-offs or ‘levels of significance’ are established by researchers, ideally before starting their work, to later determine whether their results are ‘statistically significant’. The most commonly used threshold in research is 5% (or p<0.05). The interpretation of results using this method is quite straightforward.
For example…
If the p-value corresponding to the difference in mean weight between two groups of participants is less than this threshold (e.g. p=0.03), then we can reject the null hypothesis (i.e. that no difference exists between the comparison groups) and our result is deemed ‘statistically significant’. If the p-value is above the threshold (e.g. p=0.10), we cannot reject the null and our result is ‘not statistically significant’. This procedure is known as statistical hypothesis testing.
I want to emphasize that this p<0.05 cut-off is entirely arbitrary and there is nothing inherently special about it. It is clear that p=0.04999 and p=0.05001 are essentially the same thing, and yet the former allows you to use the magic words ‘statistically significant’ to describe your results and the latter does not. Sometimes, results that do not meet this threshold are never published because they are deemed ‘uninteresting’ or ‘unimportant’ and this has led to publication bias and p-hacking [1,2].
But what is the actual difference in mean weight between the two groups of participants? Neither the p-values nor the dichotomous (yes/no) statements regarding ‘statistical significance’ give us any quantitative information about that. And this is exactly the problem: p-values only tell us something about how likely we would obtain the same study results simply due to chance. We know nothing about the actual point estimate (here, mean weight difference) in which we are actually interested.
Say we find that the difference in mean weight between the two groups to be 0.1kg and we find this difference is statistically significant (p=0.03). This means the average weight in group 1 is different than the average weight in group 2 and that this little discrepancy is probably not due to chance (based on the p-value). Even though this result is statistically significant, is it really meaningful?
The answer to that question entirely depends on the context and is up for interpretation. If we are running a randomized controlled trial with adult participants, a difference of 0.1kg in mean weight between the two groups is probably not important, even though it is ‘statistically significant’. However, we would certainly feel differently if we used a different group of participants; 0.1kg would be a much more meaningful difference in two groups of neonatal infants, for example.
When we increase the number of outcomes, we become more precise in our estimation of the true difference. In other words, we become more confident that our results are not just due to chance. Theoretically, if there are sufficient outcome events, even the most minuscule, meaningless differences can become statistically significant. On the other hand, an over-reliance on statistical significance can lead us to overlook important results or falsely classify uncertain results as negative ones. Because of the relationship between p-value and sample size, this problem is especially prevalent in studies with fewer outcomes. For this reason, it is crucial not to confuse statistical significance with importance.
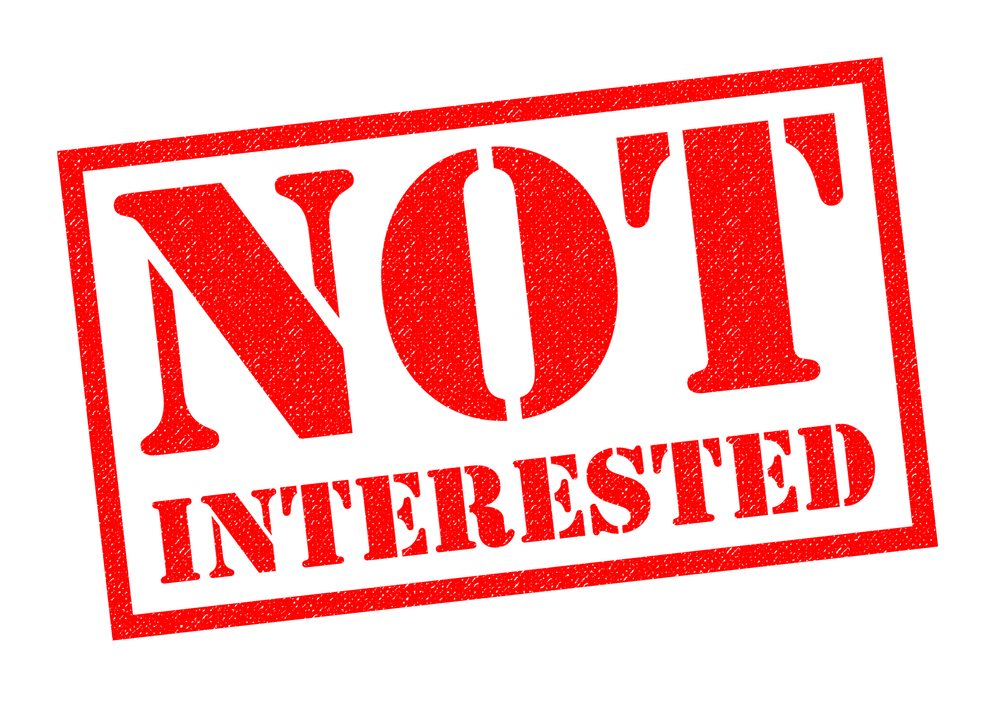
Sometimes, results that are not ‘statistically significant’ are never published because they are deemed ‘uninteresting’ or ‘unimportant’ and this has led to publication bias and p-hacking.
Let’s walk through another example to illustrate this point…
We need to decide whether a new surgical intervention is more appropriate for a cancer patient with a brain tumour compared to the standard chemotherapy treatment, based on the following hypothetical results:
Hypothetical result 1: “The new surgical intervention statistically significantly reduced the number of patient deaths compared to the current standard chemotherapy treatment (p=0.04)”
- What is significant? Do the authors mean considerably fewer deaths or just statistically significant fewer deaths? It is not clear, but possibly just the latter, since the p-value is reported. Here, we don’t have any information on exactly how superior the new surgical intervention was compared to chemotherapy.
Hypothetical result 2: “The new surgical intervention statistically significantly reduced the number of patient deaths compared to the current standard chemotherapy treatment (p=0.04). After five years, there were two fewer deaths in the intervention group.”
- Now the exact difference in number of deaths is provided and it is clear the word ‘significantly’ refers to statistical significance in this case, since two is not a large difference. As a reader, it is now important to contextualize these results. In an exploratory study in which each group had only 10 participants, two fewer deaths in the intervention group would be meaningful and warrant further investigation. In a large clinical trial with 1000 participants in each group, two fewer deaths, even if statistically significant, is less impressive. In this case, I would consider the two interventions more or less equal and base my treatment decision on other factors.
Hypothetical result 3: “The new surgical intervention did not statistically significantly reduce the number of patient deaths compared to the current standard chemotherapy treatment (p=0.07). After five years, there was 1 death in the surgical intervention group and 9 deaths in the standard chemotherapy group.”
- This time we have a statistically non–significant result that corresponds to a seemingly large point estimate (8 fewer deaths). In this case, it appears the treatment has an important effect but perhaps the study lacks sufficient power for this difference to be statistically significant. Again, we need more information about the size of the trial to contextualize the results. To simply conclude by virtue of statistical hypothesis testing that this study shows no difference between groups would seem inappropriate.
Summary
Hopefully after considering these examples, it is clear that the word ‘significant’ in the scientific context can be rather misleading. Thankfully, there are a host of other words (‘important’, ‘meaningful’, ‘interesting’, etc.) you can use instead to draw attention to the importance of your findings without referring to this arbitrary cut-off. Furthermore, when presenting results, consider using point estimates and confidence intervals instead of relying on the misleading p-value and the dichotomous concept of hypothesis testing.
Learning resources which further explain why saying that a difference is statistically significant or that it is not statistically significant can be misleading
Read the rest of the blogs in the series here
Testing Treatments
References
- Masicampo EJ, Lalande DR. A peculiar prevalence of p values just below .05. The Quarterly Journal of Experimental Psychology. 2012;65(11):22719.
- Head ML, Holman L, Lanfear R, Kahn AT, Jennions MD. The Extent and Consequences of P-Hacking in Science. PLoS Biol. 2015;13(3):e1002106.
No Comments on Don’t confuse “statistical significance” with “importance”
“Because of the relationship between p-value and sample size, this problem is especially prevalent in studies with fewer outcomes.” Why is this problem especially prevalent in studies with fewer outcomes rather than more outcomes? The entire paragraph was talking about how increasing outcomes leads to minuscule differences being “significant” but then it shifts almost randomly to talking about fewer outcomes.
13th April 2020 at 4:59 am