How certain is the evidence?
Posted on 1st June 2018 by Bethan Copsey
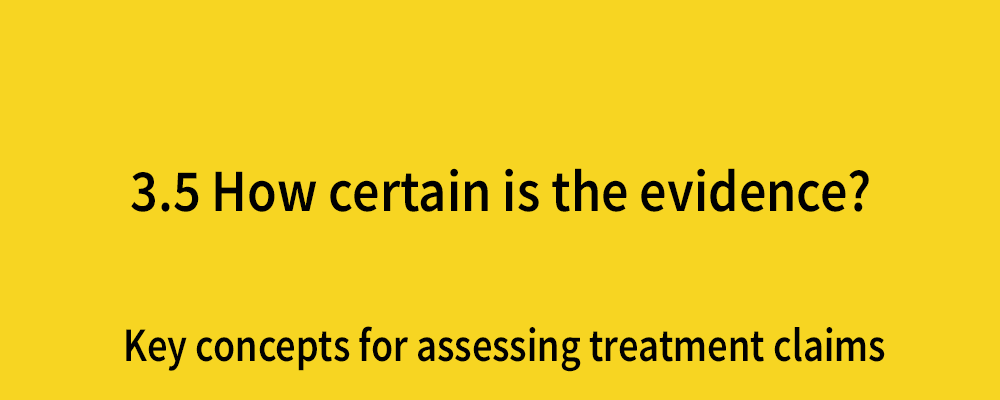
This is the thirty-fifth blog in a series of 36 blogs based on a list of ‘Key Concepts’ developed by an Informed Health Choices project team. Each blog will explain one Key Concept that we need to understand to be able to assess treatment claims.
When using evidence to inform clinical decision-making, it is important that we know whether the evidence is of good or poor quality (was the research done well?) and the certainty of the evidence (to what extent do the results indicate the likely effect of the treatment in our situation?).
In order to change clinical practice – i.e. to start using a new treatment or stop using an old one – we would like to base our decisions on high-quality evidence and to feel certain about the results of that evidence.
Pyramid of evidence types:
Among the top of the evidence-based medicine pyramid are systematic reviews. Systematic reviews synthesise evidence from all of the relevant studies in the area.
Systematic reviews are considered to be amongst the ‘gold standard’ of evidence. However, a systematic review may reveal that the evidence is uncertain. The GRADE tool was developed to help people assess the certainty of the evidence in a systematic review, and the strength of recommendations. This blog only looks at the GRADE tool for rating certainty of evidence but other tools are available too.
How to ‘GRADE’ the evidence from a systematic review?
The GRADE tool looks at the studies included in a systematic review and the overall results to give a rating for how strong the evidence is. This is separate to the findings in terms of treatment effectiveness. The review findings start with a rating of ⊕⊕⊕⊕ (like a movie with 4 out of 4 stars). Evidence can then be down-graded (or lose ‘stars’) based on:
- Limitations in the design of the included studies
- Indirectness of the evidence
- Inconsistency
- Imprecision
- Publication bias
1. Limitations in study design: Were the included studies carried out properly?
Although RCTs are considered ‘strong evidence’, they may not be carried out well. In some cases, it is possible that a well-conducted observational study can provide better certainty evidence than a poorly conducted RCT. For example, a RCT may be considered to be at high risk of bias if participants are not blinded (as knowing which treatment they received could affect their response), or if lots of participants dropped out during the trial (as this could have affected the results). It is important to remember that the only thing unique to a RCT is random allocation. Both of the biases mentioned here can apply in non-randomized cohort studies too.
To look more at the many types of bias that can occur, have a look at the Catalogue of Bias.
The most common way to appraise included studies is the Cochrane risk of bias tool.
A well-known saying in systematic reviews is: Garbage In = Garbage Out
If the studies you are including are not high certainty, then combining the results will not give you strong conclusions.
Downgrade by ⊕ – Downgrade by 1 if you have lots of unclear or high risk of bias studies, where the limitations lower your confidence in the study results.
Downgrade by ⊕⊕ – Downgrade by 2 if most included studies are high risk of bias and there are very serious limitations.
2. Indirectness: Are the included trials applicable to your situation?
Reviews may include a variety of trials which all fit the eligibility criteria. For example, in a review looking at the effects of a drug on exercise performance, many of the studies may include only children or only elite athletes, but you may be most interested in the effects on the general population. Indirectness may also come from the intervention. For instance, if in most trials, psychological treatment was often delivered for 10 hours per week in inpatient centres, this may not be relevant if you are more interested in the application of the intervention in community settings.
Indirectness is common in areas where there is little research being produced, so the reviewers may look for evidence from outside their specific area to help answer the question of interest.
Do not downgrade if all of the trials seem relevant and similar in key features which would likely impact the treatment effect. There may be one or two small trials which are indirect, which would not have a large impact on the overall findings.
Downgrade by ⊕ – Downgrade by 1 if you think that indirectness of included studies (from intervention, population, etc.) may cause differences in the treatment effects which could affect the summary results.
3. Inconsistency: Do different studies give similar results?
Ideally, we want all of the included studies to show a similar treatment effect in size and direction. If the results are inconsistent across studies, this could lead to the evidence being downgraded. This inconsistency in the treatment effect is called heterogeneity.
For example, if some studies show the treatment is beneficial and others show it is harmful, we would be sceptical about the pooled results. How do we know which effect will occur in our patient population? Even if all studies show the treatment is better than the control, we may still be concerned if some studies show a very small benefit and others show a very large benefit.
Inconsistency can be seen easily in a forest plot or using the I2 statistic. Sometimes this inconsistency can be explained, for instance, if the intervention is only beneficial in one subgroup of patients or when high doses are used.
Downgrade by ⊕ – Downgrade by 1 if the results in different studies are inconsistent and no reason is given to explain the differences in treatment effect.
4. Imprecision: Does it include enough participants?
If the results of the included studies are imprecise, this could downgrade the results. Imprecision essentially means that the estimated effect of the treatment is very imprecise; the treatment may have a large or small benefit, for instance. This would be shown by wide confidence intervals in the results and is usually because studies have included too few events or outcomes. Read more about this in the S4BE blogs about power: No power no evidence and Sample size matters even more than you think.
Even if the included studies are quite small, they may give precise results once combined together (if they have similar findings). So as well as the number of participants included in each individual study, look at the confidence intervals and total number of participants for the pooled results in the review, usually given in a ‘summary of findings’ table.
Downgrade by ⊕ – Downgrade by 1 if you feel the results are not precise enough. This is usually when too few participants are included or if the confidence interval includes both a large effect (in either direction) and ‘no effect’.
5. Publication bias: Do you suspect some studies might be missing?
The evidence would also be weakened by publication bias. This is where studies or outcomes are not published – the results of these studies could have altered the overall conclusions if they had been included in the review. It is often the case that studies go unpublished because they show no statistically significant benefit from the treatment.
There are ongoing efforts to encourage publication of every clinical trial to avoid this problem. Take a look at the AllTrials campaign which aims to do just that!
In systematic reviews, the possibility of publication bias can be explored using funnel plots. However, it is difficult to check for publication bias if the review does not include many studies. Publication bias can also be avoided or minimized if the reviewers say they searched ‘grey literature’, did a systematic search of multiple databases and did not restrict their search language.
Downgrade by ⊕ – Downgrade by 1 if you suspect publication bias.
Summary
After you have checked each factor, you need to count the number of downgrades. The review starts with ⊕⊕⊕⊕ and loses one ⊕ for each downgrade. This gives the rating for the certainty of the evidence:
⊕⊕⊕⊕ = High certainty
⊕⊕⊕ = Moderate certainty
⊕⊕ = Low certainty
⊕ = Very low certainty
If the evidence is low or very low certainty, we should be concerned about using this evidence alone to inform our clinical decision making. We want our evidence to be as high certainty as possible.
Important things to bear in mind:
- When you use the findings of a systematic review to inform your decisions, check the overall result but also remember to check the certainty of the evidence! Someone may decide not to use or pay for a treatment if the certainty of evidence is low or very low.
- The GRADE score relates to the findings of a systematic review on one treatment outcome. For example, a review could have high certainty evidence for the outcome of pain but low certainty evidence for the outcome of quality of life.
- When deciding whether to use an intervention, the treatment effect and certainty of evidence show you the benefits, but might not show you the costs. To make a fully informed decision, we should also consider costs including financial costs, potential side-effects or risks of treatment.
- This blog has focused on using the GRADE approach for reviews of interventions studies. Note that the GRADE approach is not applicable for other studies, such as those examining diagnostic tests.
Useful link:
Guyatt G, Oxman AD, Akl EA, et al. GRADE guidelines: 1. Introduction-GRADE evidence profiles and summary of findings tables. J Clin Epidemiol. 2011;64(4):383- 394. doi: 10.1016/j.jclinepi.2010.04.026