Defining bias
Posted on 9th July 2015 by Dabean Faraj
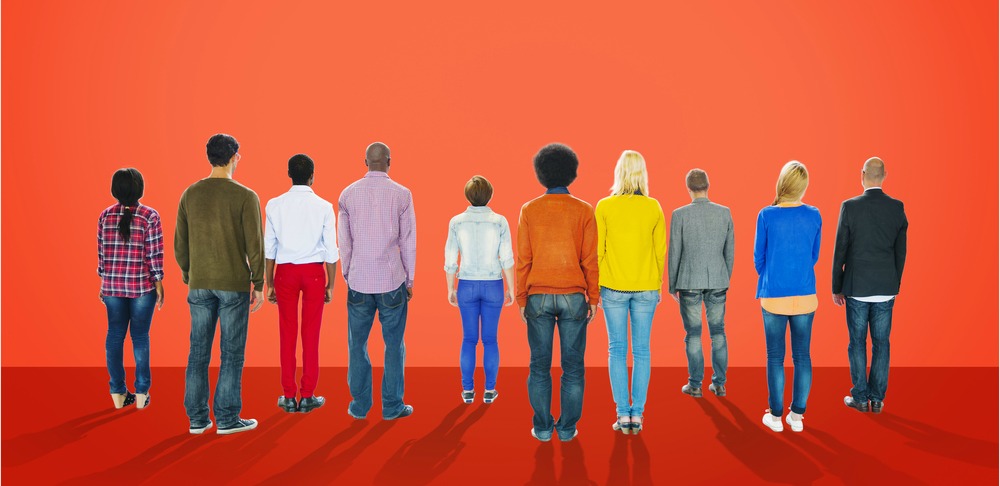
Define the following
A) Bias
B) Attrition Bias
C) Detection Bias
Introduction
Bias. A word that is so commonly uttered and everyone knows what it means. But if I were to ask you to specifically define the term, many people would struggle despite their understanding of the concept. In this blog, I hope to shine some light on the subject.
A) Bias
Well bias is not a good thing in statistics. On the most fundamental level, bias concerns the data, the results and the true value:
- Data – what you collected
- Results – what your data shows
- True Value – the actual value of whatever your trying to measure
Now in reality, the ‘true value’ of something can never be known. But statistical measures can be used to estimate the true value as best they can. ‘Bias’ is when the data you collected is skewed in some way, and this affects the results.
The truth and the problem
The truth – there is no statistical test or method of data collection that can be 100% perfect (bias free). There will always be bias. But the whole point of a good statistical test is to try and get as close to the true value as you possibly can, and you do this by reducing as much bias as possible.
The problem – there are loads of different types of bias. Loads! Which makes everything a little tricky.
B) Attrition Bias
This is the type of bias you get when you lose people in a trial your carrying out. Lets say you want to test a new type of cancer medication. Imagine you give half of your participants the new medication, and the other half the current medication/placebo. As you follow your participants along, some people will drop out of the trial along the way. Often, this is because they find that their treatment isn’t working for them, so they don’t want to continue.
Now you can respond to this in a couple of ways. One of which is to completely ignore any of the data of the people who dropped out – as if they were never in your study. However, this may cause a problem because it may be that those who dropped out, did so because they didn’t find the medication effective and the whole point of your study was to test if the new drug was more effective than current treatment/placebo. This is a problem!
C) Detection Bias
This type of bias is related to the method of ‘blinding’ used in trials. Lets continue with our cancer medication trial. A good study would make it so that
- the patients don’t know which drug they are taking
- the examiners don’t know which drug the patient has taken. So they don’t know which outcome they are measuring.
BUT! Blinding only works to an extent. Because it may be such that even though these measures have been used to conceal any knowledge of what they are taking/measuring, they may still know. Perhaps the examiners are so familiar with the symptom profile of the old cancer medication, that when they assess the patients, they know immediately that it must be the old medication that they are taking. So they therefore may act differently to patients who don’t show the same patterns. This is a problem!
Links
Really useful: http://bmg.cochrane.org/assessing-risk-bias-included-studies
Also, check out Katherine’s blog on another type of bias, Language Bias. You can also read other S4BE blogs about bias, here.
No Comments on Defining bias