No power, no evidence!
Posted on 21st January 2014 by Maarten Jansen
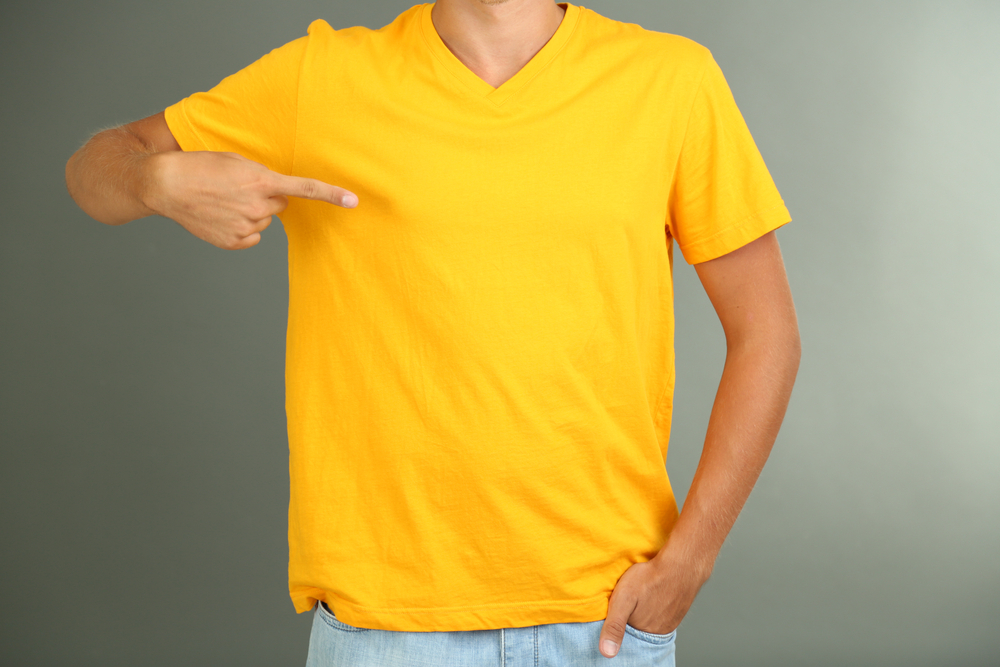
Power can mean different things in different worlds. When I think of power in general, ‘influence’ and ‘playing games’ immediately pop up. However… there’s definitely more to power than those two examples. In scientific studies, a test must have enough power to detect an effect when there is one in reality (the evidence). If a test has no power to detect the effect you anticipate, you know the answer to your test before you start collecting data.
Let’s assume there is a general practitioner who sometimes wears very bright yellow shirts. This general practitioner in particular happens to treat many severe migraine patients. During his career he starts to notice that people he treats while wearing one of his bright yellow shirts, seem to have less pain during the rest of their migraine attack, how exciting! Let’s assume from this point on that there actually is an effect of wearing bright yellow shirts when treating patients who are experiencing a severe migraine attack.
All excited, he starts his own little experiment and decides to treat his next three migraine patients while wearing a bright yellow shirt and selects three other migraine patients who he treats in a plain black shirt. So, he aims to test if the patients who were exposed to his weird fondness for yellow shirts experienced less pain during the rest of their migraine attack than those who were not exposed. He calls all six migraine patients after five days to ask them how painful their migraines were in the period after the consultation, on a pain-scale from 1 to 5 while allowing them to choose only whole numbers (1,2, 3, 4 or 5).
“Want to be mellow? Look at my yellow!”, he thought. “The test will definitely prove that patients with migraines are better off after seeing one of my beautiful yellow shirts than those who did not.”
Unfortunately he soon discovered that both groups on average perceived their pain scores to be a 4 on the pain-scale. All disappointed, he started to whisper to himself: “I guess yellow doesn’t make them mellow at all.. right?”
Well, he is correct that currently his experiment did not produce any evidence to support his hypothesis. You may however have some questions about the setup of his experiment. Was the experimental setup powerful enough to capture the effect of seeing a bright yellow shirt on the perceived pain during a migraine attack?
Effect size
The first thing to note is the effect size he anticipated. Considering the severity of a migraine attack, a change from 4.5 to 3.5 on the pain scale of 1 to 5 is quite relevant (I would say!). The used pain-scale was not able to measure this (possible) effect since he used a scale that likely results in people choosing a pain rate of 4 in both cases. This demonstrates that he could better have chosen a scale that goes from 1 to 10, allowing people to choose a 7 and a 9. This also allows me to demonstrate that bigger effects are easier to detect! If wearing a yellow shirt caused a reduction from 4.5 to 1.5 on the pain-scale, it is more easily detected, since they would be likely to choose a 4 and a 2 in this case. You therefore need to make sure your test can actually capture (or detect) a relevant difference!
Sample size
The second thing to note is the amount of people he included in his experiment, only three in both groups. It seems a little ‘weak’ when you compare it with an (extreme) situation in which he would include a total of one thousand patients per group. Only using three patients per group does not provide a clear picture of the group average pain-score. There is much room for sampling error (the difference between the true average of all migraine patients in the population and your sample average), making it hard for your test to identify or detect differences between the two small groups. When, in example, one thousand migraine patients were included, the picture of each group is much more clear, which makes it easier to distinguish between the two groups (reducing the influence of sampling error to mess up the results). This is the main reason why in randomized controlled trials, bigger is most often seen as better (at the consequence of being more expensive than smaller trials).
Significance level
The power of a test also depends on the minimal significance level you choose for your test. The significance level used most in literature is 5%. In our example, the null hypothesis would state that there is no difference between the two groups of migraine patients. The alternative hypothesis would state that there is a difference between the two groups. When you perform a test on your data, you test if the data supports the null hypothesis or not. In our case you would test if the data you found fits the null hypothesis (no difference) and only when it is 5% (or less) probable that you would have found your specific average (the sample) if the null hypothesis was true, you accept that the data does not back up the null hypothesis but instead favors the alternative hypothesis.
If you set your minimal significance level to 10% it is more likely that your test will find a significant result and thereby captures an effect (you sooner accept the alternative hypothesis). However, while increasing your minimal significance level up from 5% to 10% increases the chance that you will reject the null hypothesis in situations in which the null hypothesis is truly wrong (which is a good thing), it also increases the chance to reject the null hypothesis when it is in fact right (which is a bad thing) you make the wrong choice!
Too much power?
Too much power never is a good thing! Since you don’t know if there in reality is a true effect, you must avoid making your test excessively powerful! If you increase the sample size to infinity (hypothetically speaking) then every difference between groups will show to be significant, no matter how small or irrelevant!
Conclusions
I hope it is clear to you that you must consider how you design your experiment to provide it with sufficient power! The most important three factors that influence the power of a test are the sample size, anticipated effect size and significance level of your test. You might then ask yourself: how do I choose all of these parameters? Well… if you know some of the variables (e.g. anticipated effect size and the significance level) than you can choose to browse the internet for sample size calculators at a certain power level (normally a power level of minimally 0.8 is considered best but that’s a different story) to complete your experimental setup!
Happy testing!
Reference
Park, Hun Myoung. 2008. Hypothesis Testing and Statistical Power of a Test. Working Paper. The University Information Technology Services (UITS) Center for Statistical and Mathematical Computing, Indiana University.” https://scholarworks.iu.edu/dspace/handle/2022/19738
No Comments on No power, no evidence!