Efficacy of drugs: 3 examples to get you to truly understand Number Needed to Treat (NNT)
Posted on 15th April 2016 by Tran Quang Hung
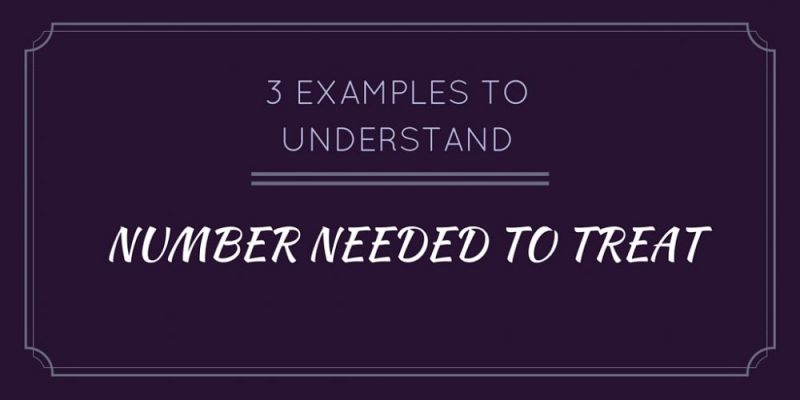
Come with me.
I’ll show you the best way to display the efficacy of a drug.
No difficult percentage. No complicated proportion. Just a plain number. And lots of interesting information behind it.
Its name is Number needed to treat (NNT).
We’ll go through 3 examples to gain a deep insight into this cool statistic.
Ready to go?
Example 1: What is number needed to treat?
Imagine that in the year 2100, our world is affected by a weird syndrome, called syndrome Bizbuzbaz (terrible name, isn’t it?). It causes patients to easily lose self-confidence, to lose hope in others. Patients give up chasing their dreams, walk away from loved ones, and live in depression. Here, there and everywhere, lots of people are affected. Death rate is so high.
“Hey, don’t worry. I’ve got SuperMed for you. It will help you cure syndrome Bizbuzbaz”, says a pharmacist. “The NNT is 2.”
While you’re still wondering “What does an NNT of 2 mean?”, the pharmacist rapidly draws a graph on paper:
“We conducted a randomized trial to test the efficacy of SuperMed. There were 2 groups: a group treated with SuperMed and a group treated with placebo. 100 patients in each.
In the group treated with SuperMed, 75% patients were still alive after a 1-year course. In the group treated with placebo, only 25% patients survived.
So, you can see 25% patients don’t die regardless of the lack of a “real” drug. 25% will die, even if they get an effective drug. SuperMed helps 50% of patients survive. It means that for every two patients, SuperMed prevents one death.”
The pharmacist stops speaking. That’s when you start to get the point.
Brief synopsis:
(1) Many people will never experience a bad outcome, regardless of risk (which makes it impossible to prevent one).
(2) For some people, the treatment simply does not work.
(3) Some people will benefit from an effective drug. We will derive an NNT based on the percentage of this group of population.
The thing is, we don’t know which of these three scenarios will apply to a given patient. So, we must treat all patients, and hope that they all fall under either (1) or (3).
Example 2: The comparator counts
I have a confession to make.
The interpretation of NNT in story 1’s example is not correct. Well, actually, it’s not wrong, but insufficient. The explanation should be: “NNT means that for every two patients, SuperMed prevents one additional death, in comparison to placebo.” And the brief synopsis above is only correct when the comparator is placebo.
You may wonder why I must make that clear. Well, take a look at the example below, and you’ll understand the reason.
In this study into the efficacy of oral anticoagulants and aspirin in nonvalvular atrial fibrillation, the NNT of anticoagulants (medicines that help prevent blood clots) is 30, for high-risk patients [1].
Wait, what? Only 1 in every 30 high-risk patients gets benefit from oral anticoagulants?
No, the statement should be: 1 in every 30 high-risk patients gets additional benefit from oral anticoagulants, compared with aspirin.
This study talks about the efficacy of oral anticoagulants when compared with aspirin. So we have to take aspirin into account when interpreting the NNT of oral anticoagulants.
Let’s take a look at the demonstrative image below.
The above graph shows the results for the high-risk group.
So, for this high-risk group:
- 94.1% patients (100% – 5.9%) won’t be affected by stroke, no matter whether they use aspirin or oral anticoagulants;
- 2.6% patients will be affected by stroke, no matter whether they use aspirin or oral anticoagulants;
- Oral anticoagulants prevent stroke in an additional 3.3% patients, in comparison to aspirin (5.9% – 2.6%). So, the NNT is 30 (i.e. 3.3% = 1/30) [1].
For the low-risk group, the authors reported that NNT of oral anticoagulants is very high (“NNT not significant”) [1]. This means that the effectiveness of oral anticoagulants is roughly the same as aspirin in this group of patients.
Brief synopsis:
(1) The number needed to treat (NNT) is defined as the expected number of people who need to receive the experimental rather than the comparator intervention for one additional person to either incur or avoid an event in a given time frame. Thus, for example, an NNT of 10 can be interpreted as such: ‘it is expected that one additional (or less) person will incur an event for every 10 participants receiving the experimental intervention rather than control over a given time frame’. [2]
(2) The NNT is a comparative measure of effect, not a characteristic of a single intervention, so we must figure out what the comparator is (in a lot of cases, the comparator is not a placebo).
(3) A very high NNT means that the effectiveness of the intervention in question is almost the same as the comparator.
(4) With different risk groups, we get different NNTs.
Example 3: The outcome counts
In 1995, Moore conducted a study to assess the effectiveness of the triple therapy in Helicobacter Pylori treatment, in comparison to Histamine antagonist. (Helicobacter Pylori being a stomach-related condition which can cause ulcers).
Two outcomes were proposed: (1) eradication of Helicobacter pylori after 6-10 weeks of treatment; (2) ulcers remaining cured at 1 year after 6-10 weeks of treatment.
Two NNTs were identified: 1.1 and 1.8, corresponding respectively to outcome (1) and (2).
As we can see from the example above: with different outcomes, we got different NNTs. So we must take the outcome into account when interpreting an NNT.
What if the outcome (2) in the above example is not ulcers-remaining-cured-at-1-year but ulcers-remaining-cured-at-2-years?
In that case, NNT will change, because NNT is also influenced by the time course. In an other study, statin therapy shows an NNT of 364 at 90 days (in comparison to placebo) and an NNT of 93 at 3.3 years [4].
Brief synopsis:
(1) With different outcomes, we get different NNTs.
(2) NNT varies over time.
Take home messages:
To come up with an exact interpretation of an NNT, you must gather enough information. Ask these questions:
- What is the baseline risk of patients in the study?
- What is the comparator?
- What is the outcome?
- How long does the study last?
REFERENCES
1/ Van Walraven C, Hart RG, Singer DE, et al. Oral Anticoagulants vs Aspirin in Nonvalvular Atrial Fibrillation: An Individual Patient Meta-analysis. JAMA.2002;288(19):2441-2448.
2/ Cochrane handbook for systematic reviews of intervention. Version 5.1.0 http://handbook.cochrane.org/chapter_12/12_5_2_more_about_the_number_needed_to_treat_nnt.htm
3/ McQuay HJ, Moore RA. Using numerical results from systematic reviews in clinical practice. Ann Intern Med 126: 712-720.
4/ McAlister, Finlay A. The “number needed to treat” turns 20—and continues to be used and misused. Canadian Medical Association Journal 2008;179(6):549-553.
Read more of Tran’s blogs here…
How did they determine diagnostic thresholds: the stories of anemia and diabetes
Key to statistical result interpretation: P-value in plain English
Surrogate endpoints: pitfalls of easier questions
Why should medical students know about kappa value?
No Comments on Efficacy of drugs: 3 examples to get you to truly understand Number Needed to Treat (NNT)
Nicely presented!
7th November 2016 at 12:57 amI’m very happy that you like the article. I’m a huge fan of Information Mastery. Your work inspires me a lot.
10th November 2016 at 6:17 amI think the x-axis labels on Figure 2 (ASA and OAC) are the wrong way round. Am I right?
2nd May 2016 at 6:23 pmCould you explain more clearly? I didn’t find any problems with this graph.
6th May 2016 at 12:34 pmSorry Quang, You are quite right! Events prevented with OAC.
6th May 2016 at 10:43 pm