Tricks to help you get the result you want from your study!
Posted on 9th December 2016 by Sam Marks
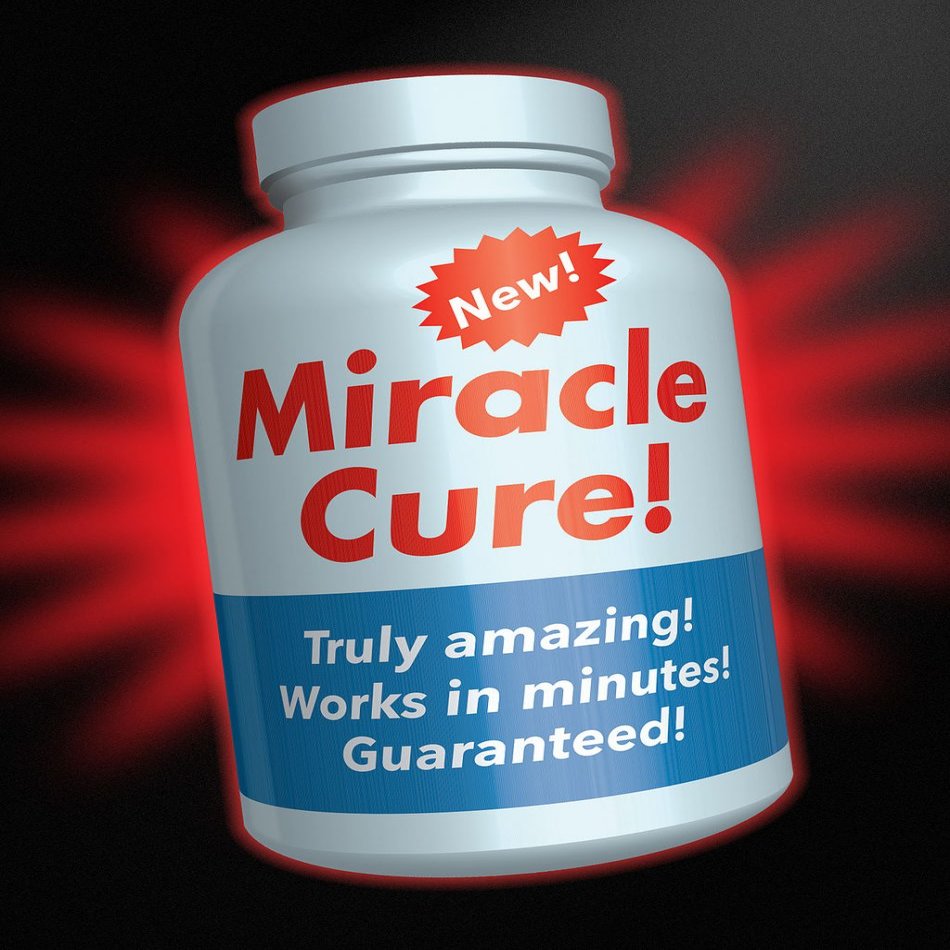
I thought about giving this blog a more sensible title. Something like “things to look out for when reading a paper on a clinical trial”. But, I needed to get your attention, didn’t I….
Let’s set the scene: An increasing number of clinical trials are funded by the pharmaceutical industry.
But this is clearly problematic. In 2012, a Cochrane Review including 49 studies, found that industry-sponsored studies testing drugs and medical devices more often favour the sponsor’s products than non-industry sponsored studies.
Mmm, sounds fishy. What might be going on here?
Well, imagine you work in the pharmaceutical industry and you have a new drug. You’ve got a lot of money riding on it, so you need to show that it’s safe and effective. You need evidence to back up your claims that this is the new best thing, and that doctors should prescribe it to their patients. The thing is, your new drug isn’t spectacular. But you really do have a lot of money riding on it.
So what can you do to make sure you get the result you want from your study?
There are many tricks you could try… (and beware, these can be fairly subtle, so even a fairly eagle-eyed reader may not notice them).
Fiddle with the study design…
- You could study the drug in a group of participants you know are likely to respond well to it. For instance, younger people are likely to respond better to medication than older people who are already on lots of medication. So test the drug in those young participants (even if they’re unlikely to be the ones who are actually prescribed the drug in real life).
- Compare your drug against another drug that’s useless. For instance, you could use an inadequate dose of the comparator drug, so that the patients receiving that drug don’t do well. Or, you could give too high a dose of the comparator drug, so that those patients experience considerable side-effects. By comparison, your drug will seem more effective and/or better tolerated.
- Timing is everything. If the difference between your drug and the comparator drug becomes significant after 2 months, stop the trial! You’ve got the results you want and you don’t want to risk the difference disappearing if you let the trial run on. On the other hand, if at 2 months your results are nearly significant, extend the trial by a couple of months, and wait until your results become significant!
Now your trial is over and you’ve done your data analysis. But wait! Despite your best efforts, using the above tricks, your results have come back negative. What can you do now to convince people that your new drug is fantastic?
Well, firstly, you could not publish your results at all. If you have enough money, you could even run the trial again and hope that next time the results will be positive.
Alternatively, you’ve got a few more tricks up your sleeve…
Fiddle with the data…
- Ignore participants who’ve dropped out of your study. People who drop out of trials are more likely to have responded poorly to the treatment they received and to have experienced side-effects. So ignore them. Including them in your analysis could show your drug in a bad light.
- ‘Clean up’ your data. You’ll probably find some ‘outliers’ in your data. An ‘outlier’ is an observation that differs markedly from your other observations (e.g. when a particular participant responds spectacularly well or spectacularly poorly to a drug, this will skew your data). These ‘outliers’ might be helping your data (i.e. making your drug look good). If so, leave that ‘outlier’ in (even if it’s likely to be false/misleading)! But if a given ‘outlier’ is not making your drug look good, remove it!
- If your results aren’t what you hoped for, go back and analyse ‘sub-groups’ within your sample. You might find that your drug worked well within a particular sub-group, such as 30-35 year old females who own 2 cats. Who cares if it’s likely to be purely by chance that this particular group seem to have responded well and if the observation itself is clinically meaningless?
- Use a different statistical test. Some statistical tests shouldn’t be used if your data doesn’t meet certain assumptions. But who cares about using an inappropriate statistical test if it means you get the result you want!
When you’re finished fiddling with your data, choose the journal you submit your study to wisely. If you’ve used any of the tricks above, a careful reader will spot them. So you’re best off submitting your paper to an obscure journal. That way, fewer people will read it and lots of them won’t read past the abstract.
Finally, why not add a bit of spin in the discussion or conclusion of your paper!
Overstate how effective your drug is. (For example, by focusing on that obscure sub-group of your sample who responded well to your drug). Understate (or completely ignore) any side-effects or harms that you might have found. A bit of embellishment won’t go amiss!
Viola! So there we have some tricks to help you get the results you want in your quest to maximise your profits.
In all seriousness, the next time you’re reading a journal article about a clinical trial, (perhaps particularly if you see the study has been funded by a pharmaceutical company), be sceptical, be critical. Look out for any of these appalling tricks.
Disclaimer: This blog is heavily based on a chapter in Ben Goldacre’s ‘Bad Science’. I felt compelled to write this blog after reading about these shocking practices in his book. If you’re as shocked as I am by the ways in which researchers and sponsors can fiddle with their studies and data, do share this blog and read Ben Goldacre’s book for much more info.
No Comments on Tricks to help you get the result you want from your study!
Hi Amit,
Thanks for commenting. You’re quite right, my views are biased and I was meaning to be deliberately provocative (as was Ben Goldacre in his book, I assume!)
Nonetheless, unfortunately I’m not as optimistic as you are. Indeed I think the results of the 2012 Cochrane Review speak for themselves: industry-sponsored studies testing drugs and medical devices more often favour the sponsor’s products than non-industry sponsored studies. Clearly something is wrong.
Nonetheless I take your points and would like to respond to them.
With reference to your 1st point, any trial which does not adhere to strict blinding & allocation concealment is at risk for this type of bias. For instance, one common mechanism for achieving random allocation of patients is for clinicians to receive envelopes. When the clinician opens this envelope, it tells them whether the next patient/participant is to enter the treatment or intervention group. There have been reports of clinicians holding up these envelopes to the light – so that they know in advance which group the next patient will be allocated to. For example, if they feel that the patient in front of them would fare particularly well in the treatment group, they may then swap envelopes to ensure that this is the case (Take a look at: http://bmcmedresmethodol.biomedcentral.com/articles/10.1186/s12874-016-0235-y)
With reference to your 2nd point, I went too far when I said ‘compare your drug against another drug that’s useless’. Comparing a drug with a completely useless comparator would be obvious. But comparing your drug with an inappropriate dosage of a comparator drug is much more subtle and difficult for regulatory agencies and readers to spot. Individuals will not necessarily pore over every aspect of the methodology section in a study. If you quickly skim an abstract for instance, would you really be asking yourself detailed questions about the dosage of the drugs being tested? And if so, would you know off the top of your head whether the dosage of the comparator drug was inappropriate (e.g. slightly too high or too low for a fair comparison)?
With reference to your 3rd, 4th and 5th points, it’s important to point out that the book that this chapter was based on was published in 2009.
You rightly point out that pre-registering, and then following, a trial protocol should mean that many of the issues raised should be avoided. However, the mandatory registering of trials is a relatively new, (and extremely welcome), requirement. Additionally, it is still not a mandatory requirement of all journals or in all countries. Where it is not a mandatory requirement, researchers/sponsors can still get away with – for example – failing to include drop-outs in the statistical analysis or ‘fishing’ for significant results.
Moreover, it’s also important to stress that systematic reviews – which aim to incorporate all eligible studies – will still be incorporating older studies which have not had to register their protocol in advance. Again, these older trials may not have had to follow a pre-registered protocol, allowing them to get away with some of these ‘tricks’. This may distort the findings of a systematic review.
Take a look at Elizabeth Loder’s blog from just last week: ‘the persistent problem of unregistered clinical trials’ http://blogs.bmj.com/bmj/2017/02/08/elizabeth-loder-the-persistent-problem-of-unregistered-clinical-trials-how-can-we-get-to-zero/
15th February 2017 at 7:59 pmBeg to differ Sam:
1. You could study the drug in a group of participants you know are likely to respond well to it- Very easily detectable by regulatory agencies. They are not fools.
2. Compare your drug against another drug that’s useless- again easily detectable by anybody practicing medicine. Compititor will sue you.
3. Timing is everything: This is why protocols are written & are registered at clinicaltrials.gov (besides with IRBs)
4. Ignore participants who’ve dropped out of your study: ITT VA per-protocol analysis
5. Clean up’ your data/ If your results aren’t what you hoped for, go back and analyse ‘sub-groups’ within your sample/ Use a different statistical test: This is why statistical analysis plan is mentioned in the protocol, before the study even takes off.
Apologies as I have not read the book you are referring, but views are biased.
15th February 2017 at 9:37 am