How much evidence is in evidence-based practice?
Posted on 26th April 2017 by Ludwig Ruf
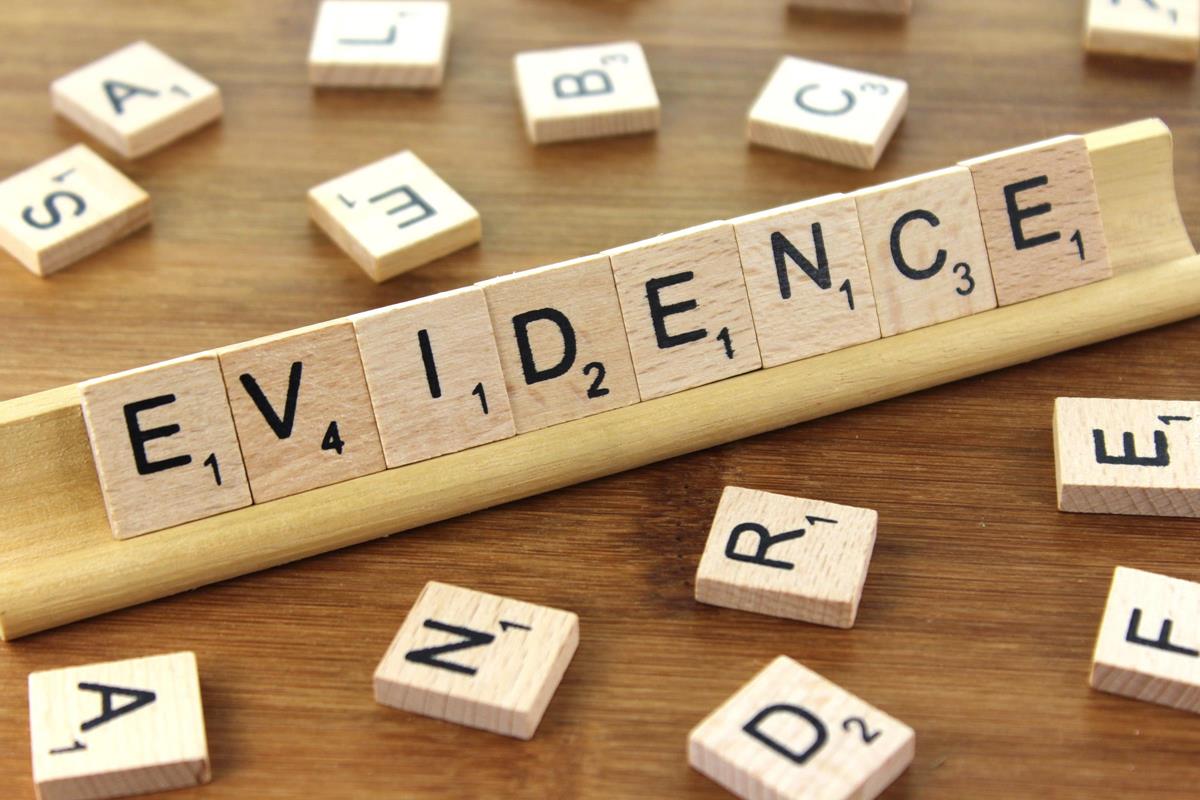
‘Evidence-based practice’, it seems, has become a trendy term…
Sometimes it feels like people claim that a given intervention/programme/policy is of good quality simply by stating that it’s based on scientific results. Now obviously the rational is straightforward: researchers seek to produce unbiased evidence while controlling as many confounding variables as possible, which practitioners can rely on. However, I think there is something missing in this equation. The question that needs to be asked in this context is: “how much can we trust published scientific literature?”
In my opinion, besides some others, two main issues need to be addressed in this context:
- Publication bias in scientific journals
- The applied statistical approaches
While I would not consider myself having much experience in either of both areas, I came across one situation recently which involved both of these problems….
The story goes as follows: a student group aims to conduct a study in epidemiology and publish the paper in an international peer-reviewed journal. Obviously, in medicine many studies are funded and many journals are open-access, so finding a low-ranked journal that has no obligatory open-access policy was the first obstacle to overcome. Having found some journals, the next obstacles were due to the fact that the group of students had only a small sample size (250 vs >100000 in some other similar studies) with results being almost consistently non-significant.
Reading through the authors’ guidelines of the selected journals was further demoralising. Some journals stated that the chances of publication are low when: a) sample size is small, b) sample size is restricted to one country, and c) results do not contribute to some novel findings. (Of course, sample size is important if a small sample means your study is underpowered. However, on the flip side, with very large samples, even trivial differences can become statistically significant). Now at this point something has to happen in the future. While the number of articles highlighting publication bias increases, overcoming the inertia of the current system will be difficult (see here, Grey matters; on the importance of publication bias in systematic reviews and Publication Bias in Psychology: A Diagnosis Based on the Correlation between Effect Size and Sample Size).
Nissen et al., 2016. The normal path to publication, successfully publishing a positive result is more likely than a publishing data failing to support a previous established claim (Nissen et al., 2016)
We know that journals are more likely to publish results that are novel and positive rather than studies claiming “nothing new under the sun” or that “taking the medication xyz has no effect on morbidity and mortality rate” (see the image above)
But those missing papers are needed to ensure that the view we have on any given topic is not distorted and thus to enable practitioners to make informed decisions rather than providing them with information from only one side of the coin. For academics on the other side, those studies would send out the message “do not waste your time and resources trying to replicate this initial finding, we can say with enough confidence that it doesn’t work.”
Such a policy is like an invitation for manipulating data as long as they show the desired results…
If you have time, I invite you to read this article about why eating chocolate facilitates losing weight (or maybe they just fooled us). Having taken a closer look at the available studies, I noticed that despite being statistically significant, the clinical meaningfulness was close to 0. How can that be? Simple maths can help in this instance to detect the issue. In plain language, the bigger your sample the higher the likelihood of obtaining a significant result even though your observed difference/change (and standard deviation) between groups stays the same. I applied this to our sample and with just 700 more participants we would have obtained a significant result (remember that there were studies with >200000 participants, so significant results are no miracle).
More important for practitioners is not whether there is a difference, but rather how big or important the difference is…
..or how much more likely someone is to get affected when being exposed to a risk factor, for example. It turned out that the majority of studies observed odds ratios of approximately 1.1. (Not entirely correct but for the sake of simplicity this means that chances are 10% higher in suffering from the disease when being exposed to the risk factor). Considering a baseline prevalence rate of 30%, the new probability increases by 3% to 33%, or put in other words, 3 more people out of 100 are going to suffer from the disease while being exposed to the medication. Surely, the bigger the sample the smaller the observed effect, but the term statistical significance is misleading in this instance.
Note that, in the majority of 1000 randomly selected published papers in the area of psychology, a p-value right below 0.05 was reported (a z score of 1.96 equals p = 0.05) (Kühberger et al., 2014)
Referring back to actual topic of the blog; unfortunately, the majority of research that has been done so far is based on traditional statistical methods, i.e. null-hypothesis significance testing (p < 0.05 = happy days, p > 0.05 = data are useless). Luckily there are researchers and practitioners (among others, Will Hopkins and Martin Buchheit influenced me the most) questioning and fighting against current standards in science and research. It is important to recognize that published research is not automatically free from any sort of error and cannot be used without any doubts to back up your current philosophy, approach or program. And we have to take published research with a grain of salt, given that we don’t know much work failing to support a certain claim was – and will never be – published.
The bottom line is; you can’t trust per se any scientific findings stating that they found a statistical significant result…
I would highly encourage you to read the statistics and result sections to make up your own judgement about whether or not the finding is meaningful, i.e. can the finding really be transferred to real life scenarios?
References
Kühberger, A., Fritz, A., Scherndl, T., 2014. Publication Bias in Psychology: A Diagnosis Based on the Correlation between Effect Size and Sample Size. PLOS ONE 9, e105825. doi: 10.1371/journal.pone.0105825
Nissen, S.B., Magidson, T., Gross, K., Bergstrom, C.T., 2016. Publication bias and the canonization of false facts. eLife 5, e21451. doi:10.7554/eLife.21451