Association is not the same as causation
Posted on 23rd June 2017 by John Castle
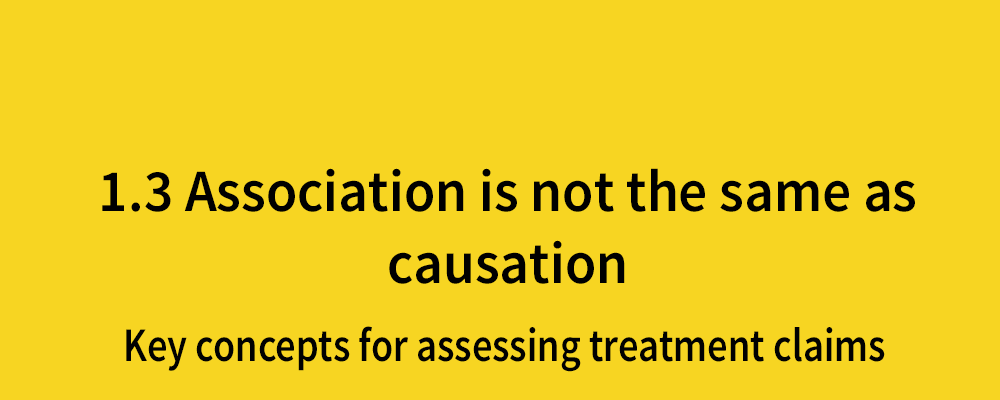
This is the third in a series of 36 blogs based on a list of ‘Key Concepts’ developed by an Informed Health Choices project team. Each blog will explain one Key Concept that we need to understand to be able to assess treatment claims.
Determining whether an outcome is directly caused by a treatment or occurs coincidentally, is an age-old problem. Often, establishing a causal link can be difficult and causation is assigned to an intervention where the evidence cannot prove this. There are many examples where association may have been mistaken for causation and it is important that when assessing the evidence of a causative effect, proper trials are conducted to rule out other variables.
Spurious correlations: Eat cheese and get tangled in your bedsheets…
There are many coincidences in life where correlations can be found between two seemingly random factors. It is unlikely that one thing causes the other yet some might believe that they are. For instance, cheese consumption in the US between 2000 and 2009 correlated with the number of deaths by entanglement of people in their bedsheets [2]. Does one of these factors cause the other? Probably not.
Spurious correlations: Watch a Nicholas Cage film and drown in a pool…
Also, the number of people who drowned in a pool between 1999 and 2009, correlated with the number of films starring Nicholas Cage that were released during that time [3]. It’s highly unlikely that Nicholas Cage is the cause of people drowning in pools (although, if victims were watching a Nicholas Cage film, they may have benefited from drowning), but the two rates are almost identically correlated.
Observational studies: Alcohol consumption and death rates
Observational studies are those which look at the rate of an outcome in groups that were differently exposed to an intervention or risk factor. They can provide strong evidence of association between factors. However, they cannot with certainty be used to prove that the factors investigated are causally linked. This is because they may not have accounted for unknown variables that affect the result.
In 1997, a very large population study looking at alcohol consumption and death rates (amongst other variables) was published by the New England Journal of Medicine [4]. It showed very clearly that moderate levels of drinking (between 1-2 drinks per day) was associated with a decrease in death rates from all causes, particularly from cardiovascular disease, even compared to people who don’t drink at all.
There is undeniably an association in their results, but we cannot say with certainty that the alcohol itself caused the increase in life expectancy. This is because there may well be other factors involved that explain the difference. For instance, what if people who have a drink a day are more relaxed? There is an association between stress and increased risk of cardiovascular disease, and the result could have been caused by this. Another possible explanation is increased social interaction in people who drink moderately, as loneliness may also be associated with shorter life expectancy [5].
Example 2: Smoking and cancer
In the first half of the 20th century it was very hard to say that cigarettes caused health problems.
Tobacco companies with conflicted interests pushed the idea that the increase in lung cancer in this period was due to increased road tarring and air pollution. One of the first men to establish the link between smoking and lung cancer was Sir Richard Doll (the real first is likely to be a German man named Fritz Lickint whose ideas were usurped by the Nazi government). Sir Doll asked patients with lung cancer many questions about their life, including their level of tobacco consumption. Strikingly, the biggest association he noticed between lung cancer rates was with tobacco consumption. This association repeatedly held, even when studying many different groups of people from multiple backgrounds, including doctors. As time went on, the amount of studies that showed this association accumulated and the collective evidence gave strong indications that lung cancer was causally related to cancer. Animal studies showed that tobacco ‘juice’ increased the rates of cancer in rats. Cellular studies showed that cigarette smoke was ‘deadening’ the tiny hair cells that line our windpipes, allowing pollutants to get in the lung. Mounting data from observational studies eventually pressured the government into recommending that people stop smoking.
This is an example of where an association may be very tightly correlated and reproducible in different populations, and so gives enough evidence for people to act. However, situations like this are rare and problems come when associations are inappropriately portrayed as causation.
The best way to prove a definitive cause, particularly for a medicine or intervention, is by conducting a randomised controlled trial.
Testing for causality in a randomised controlled trial (RCT)
A randomised controlled trial is a type of study that looks at occurrence of outcomes in different groups which are selected in such a way that confounding factors are unlikely to have an impact on the result.
Imagine factor 1 is a treatment and factor 2 is the number of people experiencing a particular symptom. Whether or not participants receive the treatment (factor 1) should be the only difference between the two groups. Ideally, everything else about the groups should be exactly the same: their age, their sex, their ethnicity, their long-standing health, the food they eat, the time they wake up, the relationships they have, absolutely everything. This way, we would know that the change in factor 2, i.e. any change in their symptoms, is brought about entirely by the effect of factor 1 not some other factor, the influence of which may be impacting the results in ways we cannot hope to imagine.
Obviously, we don’t live in an ideal world. We live in a world where everybody is different and it is impossible to ensure, with complete certainty, that no other external factor is causing a change in factor 2. To overcome this, we try to make sure that the people in each group are as similar as possible by randomising them to different groups so that the many variations between people are equally spread – effectively cancelling each other out. Then, we try to minimise the effect of external factors by ensuring that the only thing which changes between the groups is exposure to the treatment.
By controlling all factors, other than the variable we want to study, we can say with reasonable certainty that there is indeed a causative link between the two factors.
So beware of claims that an outcome is caused by a treatment…
When reading an article that says a treatment or lifestyle factor is associated with better outcomes, be wary. The people who seek and receive a treatment may be healthier and have better living conditions than those who do not. Therefore, people receiving the treatment might appear to benefit, but the difference in outcomes could be because they are healthier and have better living conditions. There are dozens of ways in which external factors can influence experimental results, even in a clinical trial.
Disentangling cause from association is a tricky business and it takes a brave person to claim that they can definitively prove one factor causes another. What you should take away from this is a healthy dose of scepticism. If you come across someone professing that one thing causes the other, assume that they’re wrong until you’re convinced otherwise. Ask: is what you have an association or a cause? How was this investigated? Was the study an RCT? How were all other variables kept the same.
When it comes to a treatment, remember that whilst the outcome of a trial may show an association between a treatment and an outcome, the treatment may not necessarily be the cause.
No Comments on Association is not the same as causation
For a brilliant, real life, worked example check out Ziff’s systematic review/meta-analysis of the relationship between digoxin and mortality.*
Many observational studies showed increased mortality in patients on digoxin compared with control. For years this put many clinicians off using it in patients with symptomatic heart failure. The overall risk ratio for digoxin was 1.76 [95% CI 1.57 to 1.97]) – i.e. implying a 76% increase in mortality, with a range of roughly half as much again to double. An increase was still evident after adjustment for risk factors (1.61 [1.31 to 1.97]), and in propensity matched studies (1.18 [1.09 to 1.26]).
However, in prospective randomised controlled trials there was no detectable difference (0.99 [0.93 to 1.05]).
This just shows that even after having apparently adjusted for the factors we understand, such statistical manipulations can rarely (ever?) be fully reliable.
A good example of forgetting this principle was the furore over Jeremy Hunt’s use of observational data about increased 30-day mortality after being admitted to hospital at the weekend. He asserted that it ‘proved’ that more doctors were needed at weekends, and fought an ugly political battle with junior doctors over it.
Subsequent, more methodologically sound, research threw considerable doubt on his explanation for the observed difference.
An observational studies vields hypothesis-generating evidence
A randomised, controlled, interventional study yields hypothesis-testing evidence
Refs
3rd July 2017 at 11:13 am====
1. http://www.bmj.com/content/351/bmj.h4451
2. http://www.cebm.net/weekend-effect-fact-fiction/
3. http://jamanetwork.com/journals/jama/article-abstract/2594727
Thank you for your feedback Jennifer. I agree with what you’ve said and have edited the article in places where I think it could be improved in retrospect. Hope you’re well. John
26th June 2017 at 11:13 amSpot on that in observational studies you can’t say correlation is causation. However, in controlled experimental studies, which are prospectively done, you can say that with reasonable certainty a treatment causes (not is associated with) an effect, if that effect was the primary outcome and a significant difference was shown. Scientists are not testing for associations in RCTs. They’re testing whether a treatment is the causative link. That is, authors do say in their conclusions this medication resulted in longer progression free survival than this medication/placebo or this medication resulted in lower mortality compared to this other medication in a certain patient population. To sum up, retrospective/observation studies = association/correlation and prospective, controlled, experimental studies = causation.
24th June 2017 at 3:27 pm