All should be followed up
Posted on 27th November 2017 by Ed Walsh
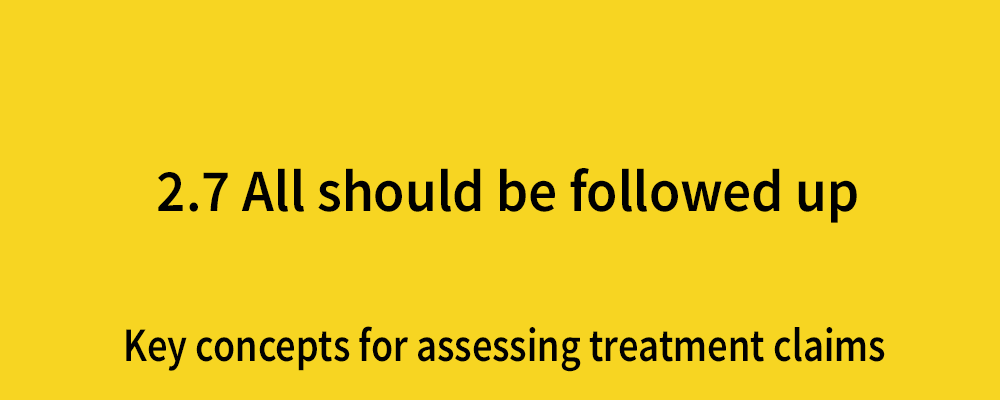
This is the nineteenth blog in a series of 36 blogs based on a list of ‘Key Concepts’ developed by an Informed Health Choices project team. Each blog will explain one Key Concept that we need to understand to be able to assess treatment claims.
People dropping out of trials or being lost to follow-up are not unusual in research, but it can lead to biased estimates of treatment effects. People drop out of trials and don’t get followed up for a multitude of reasons [1]. For example, taking part in a trial often entails a time commitment that some participants can’t maintain because of other commitments in their day-to-day life. ‘Loss to follow-up’ may occur because the research team loses contact with the people who have participated in a trial.
So, when does loss to follow-up become a problem?
Loss to follow-up becomes a problem if a lot of participants ‘go missing’, particularly if the proportion of missing participants differs between the treatment comparison groups [2]. For example, if 75% of participants from one group are missing and only 15% from a comparison group, comparing the results of the remaining participants in the two groups isn’t going to be a fair comparison.
Say you’re looking at a study comparing a new drug for headaches (named Amustriptan) with an existing drug, to assess whether the new drug is better than the existing one at reducing their headaches. Participants in the trial are asked to fill out a questionnaire before the drugs are injected and at one week, and one month after injection.
Eight percent of the people assigned to take Amustriptan failed to fill out the last questionnaire (they were lost to follow-up), compared to only 1% who failed to do so in the old drug group. The available results suggested that fewer people assigned to Amustriptan had headaches one month after treatment, compared with those assigned to the existing drug. But what about the loss to follow-up?
Misleading results?
The people in the Amustriptan group may not have filled out their one month questionnaire because the number of headaches they experienced increased, so much so that they couldn’t fill in the questionnaire because they were in bed with a headache at the time they were supposed to be filling it out. In this scenario, the 8% loss to follow-up makes the drug seem much better than it is, because those not included in the results had negative outcomes.
It can work the other way too. People in the Amustriptan group might have dropped out because of how much better they felt. They might have had no headaches at all since the injection and decided not to come back to the trial because they were feeling much better. In this instance, the 8% loss to follow-up actually makes the drug seem worse than it is, because those not included in the results had positive outcomes.
There’s a third possibility too. Occasionally, loss to follow-up doesn’t bias the results one way or the other [3]. For example, if the Amustriptan group had a higher loss to follow-up because the trial coordinator forgot to give an unselected sample of them the questionnaire a month after their injection, then the results could still be valid. This is because the loss to follow-up is random – it’s not caused by a difference between the participants who dropped out and those who didn’t.
Fighting bias with analysis
Researchers are sometimes able to counter the effects of participants dropping out or being lost to follow-up using ‘intention to treat analysis’. All this means is that everyone who was randomised in the study stays in the denominator of the analysis, regardless of what else happens after randomisation [4].
As you can see, loss to follow-up is not as simple as it first appears. Sometimes loss to follow-up makes results appear worse, sometimes it makes them appear better, and sometimes it doesn’t bias them one way or the other. Intention to treat analysis is a useful tool that researchers can employ to minimise bias associated with loss to follow-up.
As a rule of thumb, large drop-out rates usually introduce bias to results [2]. When the drop-out rate is small, the reasons that participants were lost to follow-up is key to working out how the results have been affected, and whether to trust the conclusions drawn from them [3].