Fair comparisons with few people or outcome events can be misleading
Posted on 24th April 2018 by Lewis Saunders
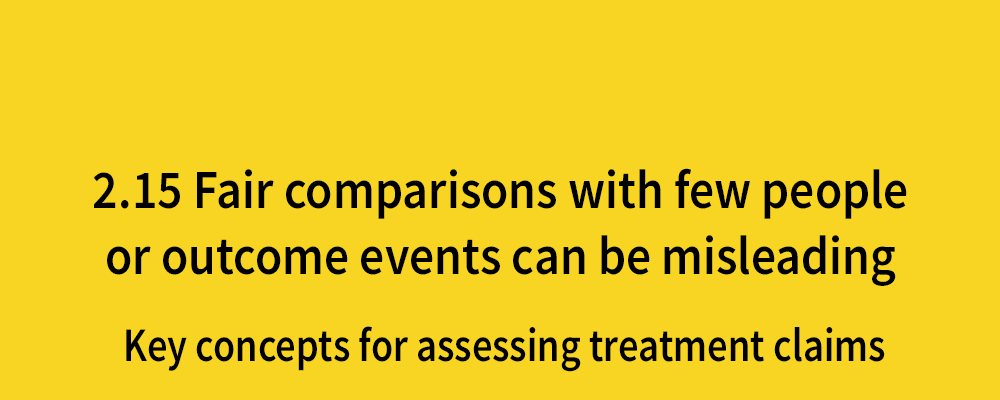
This is the twenty-seventh blog in a series of 36 blogs based on a list of ‘Key Concepts’ developed by an Informed Health Choices project team. Each blog will explain one Key Concept that we need to understand to be able to assess treatment claims.
The pharmaceutical sector is becoming progressively saturated with new medications determined to outperform their predecessors. Being able to assess the validity of interpretations made within trials is more important than ever.
In particular, the common statistical methods used to assess the effect of an intervention/drug can be misleading, even in a fair comparison, if the design of the study is inadequate. This inadequacy may relate to not having enough people recruited to the study (small sample size) to yield enough measured events (few outcome events). An example of an ‘outcome event’ is measuring how many participants in a study went on to have a heart attack after taking a particular drug.
Ultimately, it all comes back to the fundamental statistical principle of power.
A quick refresher…
Statistical significance indicates that the difference detected in comparing interventions is unlikely to be due to chance. It is evaluated using the p-value – p-values < 0.05 (5%) are often used to indicate statistical significance, whereas values ≥ 0.05 suggest that a difference may well be due to random chance.
Of interest, the American Statistician Association (ASA) Board developed a policy statement on p-values and statistical significance.
Statistical power reflects the ability of a trial or study to detect a difference within or between populations: the greater the statistical power of a study, the lower the chance of missing an effect if it was actually present. The fancy name for such an occurrence is a Type II error, the statistical equivalent to a false negative result of a diagnostic test.
We instinctively trust studies with large sample sizes, and there is good reason for this. Large study populations minimise the natural variations existing between two groups, and increase statistical power. Studies based on fair comparisons in small populations are less likely to yield sufficient numbers of outcome events and this weakens the evidence supporting/opposing a difference because random chance may be the explanation.
For example, let’s imagine a randomised controlled trial (RCT) with 10 patients in one arm and 10 in a comparison arm, with a primary outcome measure of the number of myocardial infarctions (MI) in each group. At the end of the study, there have been 6 MIs within one group, and 3 within the other group. It might be suggested that the intervention has reduced the relative risk of MI by 50%, but I’m sure you would hesitate to draw this conclusion. Why?
Hopefully, you’re thinking that the number of outcome events are too few to be able to say with any confidence that the difference reflects the differences in the treatments rather than the effects of chance. Even if each arm had 1000 participants, but the number of MIs in each group stays the same, the number of outcome events is still too low to conclude that the effects of the treatment differ. 6 in 1000 vs. 3 in 1000 is still too few to rule out chance with any confidence.
A summary of the key points:
- Studies which have a small number of outcome events, relative to the sample size, are also more likely to be influenced by random chance.
- Small sample sizes usually contribute towards low statistical power, which in turn increases the likelihood of making a Type II error (missing an effect of the intervention) and a Type I error.
- Small numbers of outcome events may exaggerate the effects of interventions.
Of course, nothing is concrete, especially in medicine. In some situations, small trials may be more suitable for the task, or may be able to generate enough statistical power. This blog is meant only to stimulate your own thinking so that you can make those decisions for yourself.
Learning resources which further explain why fair comparisons with few people or outcome events can be misleading
Read the rest of the blogs in the series here
References
[1] Haas, J. P. Sample size and power. American journal of infection control. 2012,Oct;40(8):766-767
[2] Dechartres A, Trinquart L, Boutron I, Ravaud P. Influence of trial sample size on treatment effect estimates: meta-epidemiological study. BMJ. 2013 Apr;346:f2304