Heterogeneity in meta-analysis
Posted on 31st May 2023 by Leonardo Elías Cabrera Nájera
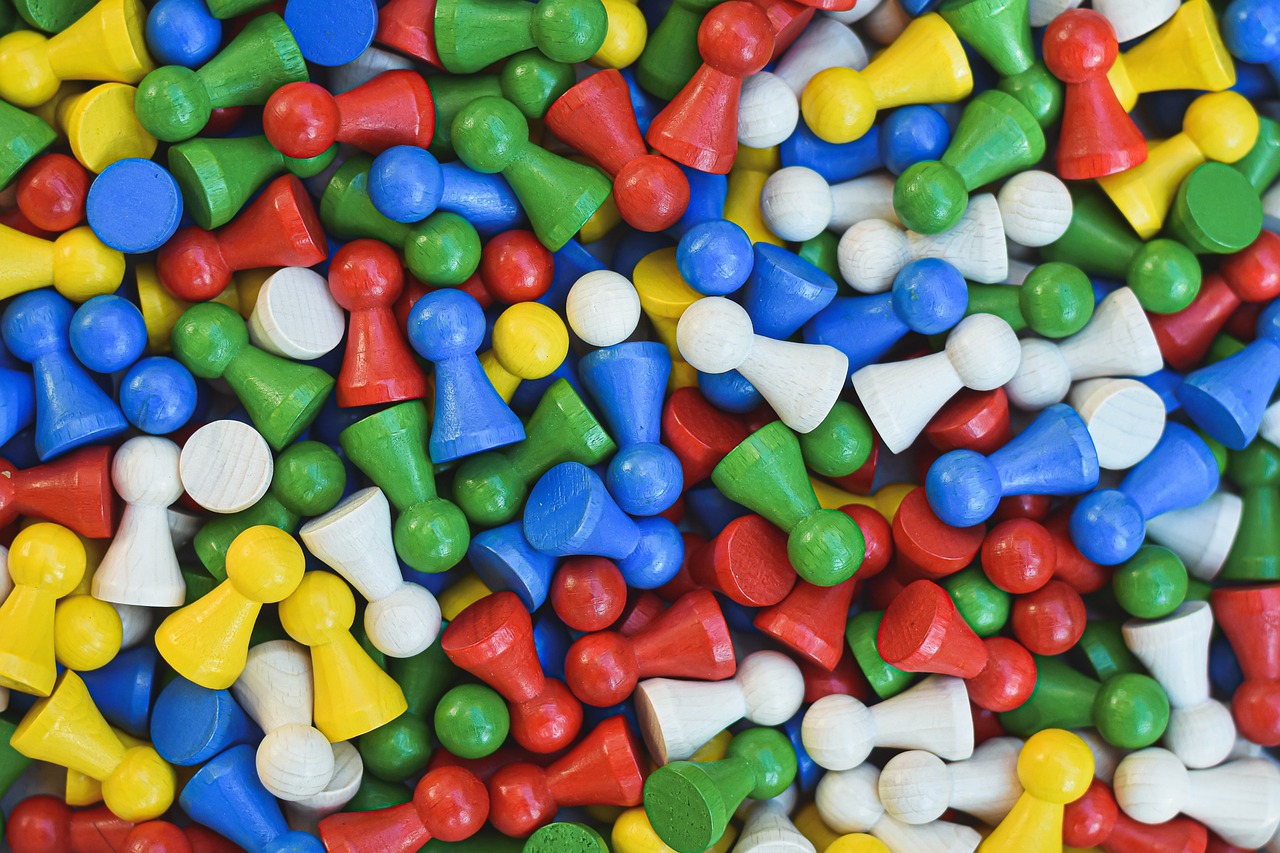
Main concepts
If you want to carry out a meta-analysis, there are some important steps to consider. In this type of statistical technique, an analysis is carried out by combining data from multiple studies. Meta-analysis (MA) is considered the pinnacle of the evidence-based pyramid. You can read about the basics of MA in this blog for beginners: Meta-analysis: What, Why and How.
To start planning, you need to know the background of each study and whether they present similarities amongst them. In a MA it is essential to consider heterogeneity, which refers to the pre-existing variability among the studies of a systematic review. The aim is to identify, measure and deal with heterogeneity as summarised in this previous S4BE blog: Heterogeneity: what is it and why does it matter?
Heterogeneity can be clinical, methodological, or statistical (Fletcher, 2007). Shown here are the different types of heterogeneity together with examples of each type:
- CLINICAL
- Participants: diverse characteristics among condition, age, gender, location
- Interventions: diverse characteristics among intensity/dose, duration, mode of administration, control
- Outcomes: variations among follow-up duration, measurement, event
- METHODOLOGICAL
- Study conduct: Variations at the level of bias, analysis, and sensitivity
- Study design: Refers to differences in random vs non-random data, crossover vs parallel, individual vs cluster
- STATISTICAL – A consequence of clinical and/or methodological diversity
- Design: the observed intervention effects are more different from each other compared to what you would expect with random error
- Outcomes: Variability in intervention effects
After analysing the different decision-making processes, it is essential to perform an analysis of the type of data and effect measures (Table 1). Some studies may be fundamental for the systematic review (SR), but due to their characteristics, they may not be able to be included in the MA being conducted. Therefore, it is necessary to first review what data is being worked with. Most MAs usually handle dichotomous or continuous data, but they are not the only types of data that exist. There are also ordinal data, counts of rare events, and time-to-event data.
Table 1. Effect Measures and Type of Data, translated from Higgins et al. (2022).
Data Type | Description | Example |
Dichotomous | Where the outcome for each person only has two possible responses | Life or death |
Continuous | Where the outcome for each person presents a numerical response (measurement or quantity). | Cholesterol levels |
Ordinal | When the result is given several ordered categories, and these are scored/summed. | Absent, low, medium, high |
Counts and Rates | Given through the count of the number of events that the individual experiences. | Number of heart attacks |
Time-to-event | When the time until the event occurs is analysed, but not all individuals in the study experience the event (censored data). | Survival time |
Understanding heterogeneity
After defining the type of data and how their effect measures will be interpreted, the next step is to determine the appropriate statistical analysis to be applied, which will depend on the characteristics of the studies. Generally, two approaches are considered: the fixed effects model and the random effects model. The fixed effects model assumes that all studies measure the same treatment effect and estimates this unique effect. It is appropriate when the studies are similar and do not present significant differences. In contrast, the random effects model assumes that the treatment effect varies between studies, estimates the average of the effects distribution, and weights by the intra-study and between-study variability. It is appropriate when the studies are diverse and may present variations.
However, it is important to understand that heterogeneity is not just a theoretical concept but also has practical implications that should be considered in the analysis. Heterogeneity can serve as an important statistical tool when interpreting the forest plot, which is a graphical display of the MA results. Cochran’s Q-test (heterogeneity test x²) is a commonly used statistical test to detect heterogeneity. However, it may lack power in meta-analyses with few studies and may identify clinically irrelevant differences. Therefore, it may not be useful when heterogeneity is inevitable.
Heterogeneity can also be identified graphically by analysing whether P < 0.10 (or 0.05), indicating the presence of heterogeneity, and whether there is a large x0 statistic in relation to its degree of freedom. Statistical measures, such as the Higgins H test (I²) and τ², can also be used to identify heterogeneity. I² measures the presence of heterogeneity as a percentage, with values close to 0 indicating homogeneous weighting of studies and values close to 100 indicating a higher degree of heterogeneity. The interpretation of I² values has an established range. If I² is greater than 70%, it is not recommended to proceed (Deeks et al., 2022).
Finally, τ² quantifies heterogeneity in random effects models, and a high value would indicate a high variation of true effects. It is important to note that this measure is not applicable in fixed effects models.
Final considerations
It is important to highlight that there are various ways to handle heterogeneity in meta-analyses. One of them is to ignore it through a fixed effects model, but this can lead to misinterpretation of the results if heterogeneity is high. Another option is to include it through the random effects model. If heterogeneity is high, it is not a cause for alarm as it can be explained. A common way to do this is through subgroup analysis, where groups are subdivided, and heterogeneity can be decreased. Other techniques such as meta-regression, control rate, covariates, among others, can also be used.
Another important point to highlight is that the article by Borenstein (2022) explains why the statistic I-squared (I²) does not provide accurate information on the variability of effect size in a meta-analysis. The argument presented is that while I² indicates heterogeneity among the studies included in the meta-analysis, it does not provide information on the magnitude of variation in the effect size. The assertion is backed up by numerical examples and graphics, which show how I² can be misleading and lead to erroneous conclusions. Therefore, we suggest that researchers and readers of meta-analysis acknowledge the limitations of I² and use alternative measures, such as standard deviation or confidence intervals, to evaluate the variability of effect size in a meta-analysis.
Feature Image by Alexa from Pixabay