Subgroup analyses may be misleading
Posted on 9th February 2018 by Ed Walsh
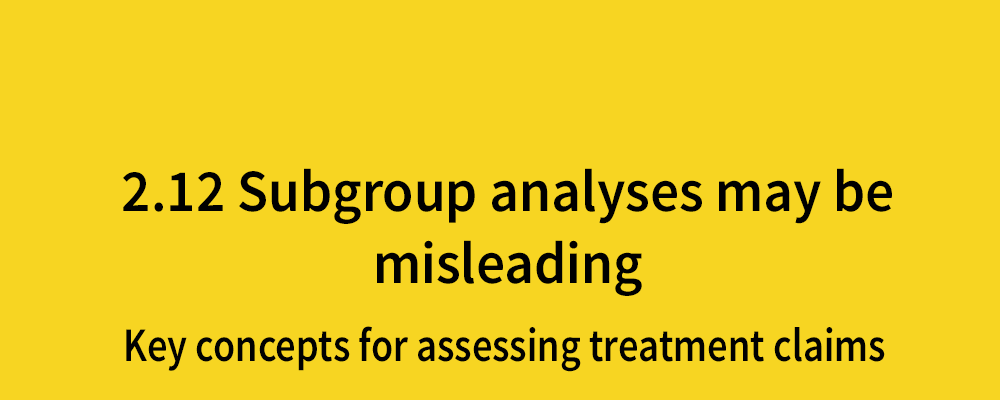
This is the twenty-fourth blog in a series of 36 blogs based on a list of ‘Key Concepts’ developed by an Informed Health Choices project team. Each blog will explain one Key Concept that we need to understand to be able to assess treatment claims.
In one well known study investigating aspirin as a treatment for heart attacks, participants with the star signs Gemini and Libra did not experience a statistically significant benefit from aspirin [1]. Aspirin reduces the chances of early death treatment following a heart attack overall [2], so why did the results from this analysis suggest otherwise?
The problem arises when we start to look at small groups within studies. Overall, aspirin was effective: it is only when they looked at small groups of participants within their study that they got misleading results. This investigation into small groups of participants with certain characteristics is known as ‘subgroup analysis’. One of the problems with subgroup analysis is the increased likelihood of a statistically significant false positive result. The more groups you investigate, the more likely you are to find a statistically significant effect by chance [3]. Let’s look at an example.
The jam sandwich trial
Imagine a trial investigating whether eating jam sandwiches improves your overall life satisfaction. 1000 people are recruited to this ground breaking new trial; 500 eat jam sandwiches every lunch- time for a week whilst the other 500 eat their normal lunch. The results are in! Disappointingly they show no overall statistically significant improvement in life satisfaction after eating lots of jam sandwiches.
The researchers decide to do some subgroup analysis to assess whether the effect of jam sandwiches is different for different types of people.
First, they looked at men and women, but neither subgroup seemed to experience any statistically significant benefit. Then they looked at people over and under 1.5m tall, but height didn’t seem to be associated with a statistically significant benefit either. They carried out subgroup analyses on weight, hairstyle, occupation, marital status, age, lung function and cholesterol level, all to no avail.
The researchers did, however, find that people with green eyes experienced a statistically significant improvement in overall life satisfaction after eating jam sandwiches daily. In reality, eye colour has no influence over the effectiveness of jam sandwiches for improving life satisfaction. It just so happened that, by chance, green-eyed participants in the jam sandwich group got a lot of satisfaction from these sandwiches compared with green-eyed participants in the control group.
False negatives
Using subgroup analysis can also lead to false negative results for some subgroups, failing to detect an effect when there is one. This happens because the groups being analysed are much smaller than in the overall study, so there aren’t enough people to enable an effect to be detected [3]. Early research based on subgroup analysis suggested that only men benefited from aspirin after a stroke to reduce the chance of another stroke or death [4]. In fact, women benefit in the same way, but the study lacked sufficient women who experienced a stroke to pick up the effect [5].
It’s worth noting that subgroup analysis, when carried out properly, can be a very useful pre-specified tool. For example, in research looking at neck surgery for people with partially blocked arteries, subgroup analysis correctly showed that various characteristics such as age, a previous stroke and heart disease, affect the risks associated with the surgery [6].
Implications
All too often, however, subgroup analyses are badly planned (or not planned at all) before research begins [7]. Sometimes effects are missed because of the small number of outcome events within each subgroup, whilst performing multiple subgroup analyses makes it much more likely any effect suggested is actually due to chance. If conclusions are made that a treatment is effective based on subgroup analysis alone, those conclusions may well be misleading.
No Comments on Subgroup analyses may be misleading
Interesting information worth sharing. Good work done
22nd February 2018 at 7:18 am