How confounding by indication can indicate problems in observational research on the effectiveness of an intervention!
Posted on 3rd December 2013 by Maarten Jansen
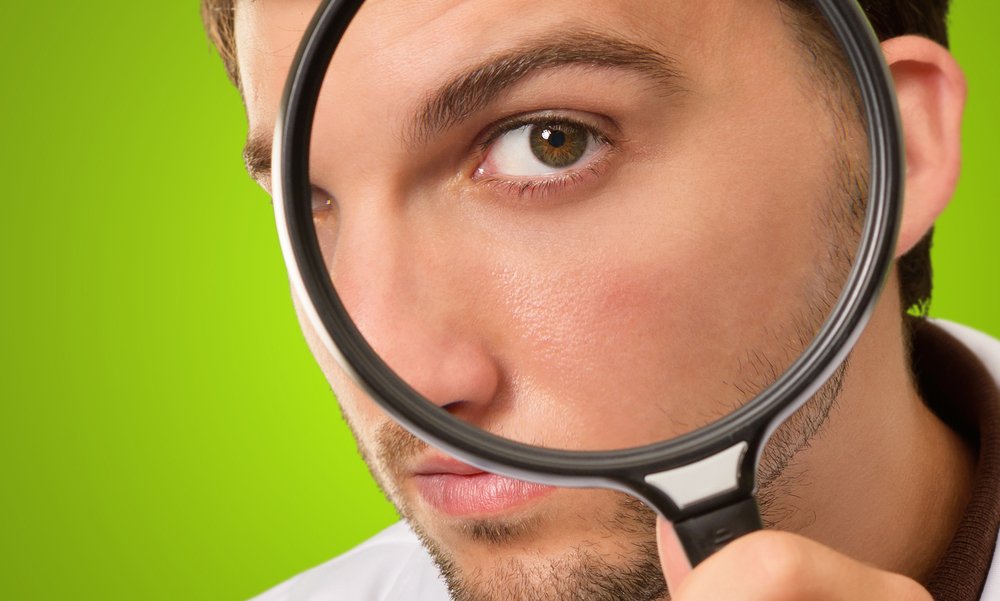
Observational research can be used to assess the effectiveness of an intervention in a non-experimental way. This is useful when assessment by a randomized controlled trial (the experimental way) is not possible or too expensive. So how does this type of study design work?
Let’s assume that, in example, a pharmaceutical company brings a nice product onto the market that promises to alleviate the symptoms of erectile dysfunction (praise the lord!) and that patients diagnosed with erectile dysfunction are monitored in a well-designed database. The database includes a relevant outcome measure in the form of how many times the person was able to… oh well…you get the point!
In addition, let’s also assume that you did not read this blog in advance when you started your research into the effectiveness of this newly marketed drug. You dig into the database and select the group of patients that received the drug upon consultation with their doctor. You also select the group of patients that did not receive this medicine and let’s assume the doctor applied watchful waiting to the second group (doing nothing and simply see if it would… rise again in due time). There is no variable in the database that explains why the doctor made his decision to either apply watchful waiting or to prescribe the drug.
Not soon after selecting the respective groups you decide to compute the vastness by which their complaints were alleviated. As it turns out, the database shows that on average, patients who did not receive drugs from their doctors experienced a vaster alleviation of their complaints (hurray for them!). You conclude that the drug performs worse than doing nothing and immediately decide to confront the pharmaceutical company with this issue!
As you enter the office of the pharmaceutical company and you confidently present your findings you think of all the people you will help by preventing their use of the drug. After your presentation there is someone with a question that goes: ‘How do you know if the lower results in the group which received our drug are actually due to using our drug?’
You suddenly feel all stiff and start to sweat… you decide to defend yourself and say ‘what else could possibly explain this?’
The person asking the question smiles and starts to explain the possibility of confounding by indication, ‘Isn’t it very likely that only patients who were experiencing a really bad case of erectile dysfunction received a prescription to start using our drug since it’s a quite severe drug to burden a patient with?’
‘So what…? Isn’t this what the drug is for?’, you said.
‘Well you see… you are right that our drugs are to be used in patients that are experiencing a really bad case of erectile dysfunction. So basically, prescription of our drug during a consult with a doctor depends on indications of really bad erectile dysfunction. In other words, the doctor is selecting only the patients that are having a really bad erectile dysfunction to use the drug.
As you may expect the prognosis of really bad erectile dysfunction is worse than that of a mild case of erectile dysfunction. You claim that the group receiving our drug, the really bad group, had worse results than the group that did not receive our drug, the mild group. This is true. However… the really bad group has a much worse prognosis than that of the mild group. To compare the results between these groups is then not legit because the chances of overcoming the erectile dysfunction in the second group are way higher than that of the first group in the first place (at baseline)! It is likely that your research is suffering from confounding by indication!
What you should have compared are groups of patients with the same chance of overcoming their erectile dysfunction, of which one group received our drug and the other group was subjected to watchful waiting. I’m sure your results will be turned around and support the use of our drug in this case as I predict that for really bad erectile dysfunction patients the drug is effective. By excluding this form of confounding by indication from your analysis you will get (more) valid results.’
The previous problem has it’s roots in the fact that we did not perform a (blinded) randomized controlled trial with randomization of patients among the “experimental” group receiving the drug and the “control group” receiving watchful waiting but instead created the groups on the basis of having used the drug or not.
In this case you missed the fact that the prescription of the drug to only the worst cases of erectile dysfunction did not create “randomized” groups but instead a group with a good chance of recovery versus a group with a bad chance of recovery. In other words, you are (wrongly) assessing the “watchfull waiting” in a group with a good natural chance of becoming better and the “drug” in a group that has a very limited chance of becoming better at all, while assuming the chances of recovery in the future are equal in both groups at baseline. This is a clear misconception!
It is likely that when a group of patients with a bad case of erectile dysfunction is split in two and one group is provided with the drug while the others simply undergo watchful waiting, the drug is effective (or at least we hope so…)!
So… what have you learned from this new experience? In order for results of observational studies into the effectiveness of an intervention to have meaning you should at least consider whether or not confounding by indication is able to influence your results and try to prevent this from influencing your conclusions that you derive from your data.
Reference:
E. Hak, Th J.M. Verheij, D.E. Grobbee, K.L. Nichol, A.W. Hoes. Theory and methods: confounding by indication in non-experimental evaluation of vaccine effectiveness: the example of prevention of influenza complications (2002). Journal of epidemiology & community health; 56: 951-955.
No Comments on How confounding by indication can indicate problems in observational research on the effectiveness of an intervention!