‘No evidence of effect’ versus ‘evidence of no effect’: how do they differ?
Posted on 9th October 2020 by Elpida Vounzoulaki
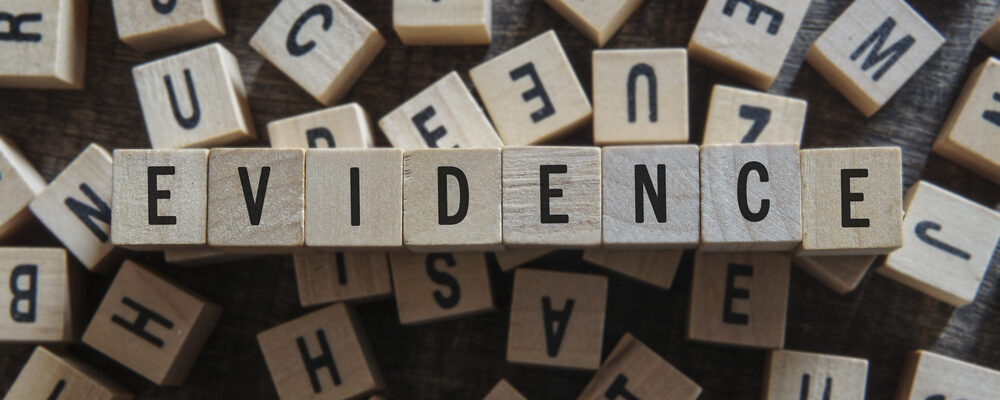
There is large debate surrounding the misrepresentation and incorrect judgement of published research results that originates from the misuse of definitive claims such as ‘no evidence of effect’ and ‘evidence of no effect’.
Claims like these are quite nuanced and often used without being completely understood.
So how does ‘no evidence of effect’ differ from ‘evidence of no effect’?
Claiming ‘no evidence of effect’ translates to suggesting that overall, the study did not manage to prove that the treatment or intervention leads to an expected outcome (e.g. no evidence that drug X can prevent heart disease).
No evidence of effect does not always mean that there is no effect in real life. This assumption is often the result of insufficient data. For example, a small study sample can lead to a lack of power to detect a real difference. (1) However, sometimes, even if a study has an adequate sample size, there are other factors influencing the results, such as study quality. (1) This should not by any means be confused with ‘evidence of no effect’.
Claiming that there is ‘evidence of no effect’ translates to suggesting that the treatment or intervention is definitely not effective. Such claims can often be dangerous or misleading when used in routine clinical practice and policy decision making.
The current situation
A study by Smith et al. aiming to investigate how often authors of Cochrane reviews made incorrect claims of ‘no difference/no effect’ found that they were much less common in reviews published in 2017 than in those published in early 2000s. (2) These findings reflect on a global effort made to minimise bias in results interpretation, however, there is still room for improvement.
Cochrane recommends avoiding strong statements such as ‘evidence of no effect’ when interpreting research results and instead relying on more general statements such as “There is insufficient data/evidence to indicate an effect of the intervention, compared with control, in terms of effects on [outcome]…” (3)
However, it must be noted that the responsibility of misinterpreting research results does not always lie with the researchers conducting the study. For example, randomised controlled clinical trials often do not show a significant difference between e.g. treatment X and treatment Y and are therefore called ‘negative.’ (1)(4) By calling these trials ‘negative’, it is implied that they have shown that there is no difference/no effect, although usually what these studies actually show is that there is insufficient evidence or an absence of evidence of a difference. (1)
Definitive claims in research: the bigger problem
However, the problem of misinterpretation in medical research lies deeper. In research studies, authors often use statistical significance testing to reach conclusions and suggest that there is ‘no difference’ e.g. between two treatments or ‘no effect’ of e.g. using a strategy for the prevention of a certain disease. (2) These strong statements are often included in the abstracts, which are the part of a paper that is mostly read by researchers and captured by the media.
While statistical significance serves its purpose, it is important to not solely base our interpretation on a p-value. In simpler words, just because statistical significance was found when comparing X against Y, that does not necessarily mean that the research has discovered something novel, or when statistical significance was not found that does not necessarily mean that there is no true difference.
Let us look at a simple example
A published randomised controlled trial did not show a significant difference between treatment X and placebo.
- So, does this mean that this treatment is not useful and effective and therefore should not be used in clinical practice?
- The answer is that this is not necessarily the truth as there is a chance that treatment X is effective, but the study could not prove that it is for a number of reasons mentioned above. (4)
A good alternative strategy to solely relying on uninformative p-values is using confidence intervals. If p-values are used in the context of statistical hypothesis testing, they should be accompanied by corresponding point estimates and confidence intervals.
Confidence intervals are particularly useful when reporting non-significant results. In the example below, which shows two ‘non-significant’ results (5) , the estimate that is accompanied by a wide confidence interval is potentially explained by a small study sample size and the possibility of random error. While it is not significant, the estimated effect seems to be around four, suggesting that it is possibly important, and re-conducting the study with a larger sample size might actually prove that. (5)
Original source Boston University School of Public Health. (5) Re-designed by Hassan Mahmudul
Definitive claims in policy making
Misinterpreting ‘no evidence of effect’ and ‘evidence of no effect’ or relying on absolute research claims solely based on a p-value can be dangerous, especially when making decisions about patients’ lives. This phenomenon has been largely observed in recommendations made by public health agencies, where decisions need to be carefully made.
Public health policy language is commonly dichotomous – which means it relies on a Yes or No, Right or Wrong answer. This often leads to research results being over-simplified or wrongly interpreted in an effort to provide public guidance that is simple and straightforward. To avoid these problems, policy makers should work closely with researchers, seek their advice, while researchers on the other hand should make clear what their research is about, avoid overstatements and acknowledge limitations.
It is extremely important to distinguish between insufficient evidence to make a policy recommendation from no evidence for it. It is also critical to question whether the absence of evidence can justify not taking action when it comes to public health matters.
Take-home message
It is extremely important for researchers to distinguish between ‘no evidence of a difference/effect’ and ‘evidence of no difference/effect’ and to use them appropriately when interpreting research results.
Therefore, they should invest some of their time to understand the difference between those terms in order to avoid misinterpreting their own research findings and to question these claims when they are made in published research.
Overall, researchers should keep in mind is that: ‘Absence of evidence is not evidence of absence’ while policy makers need to carefully consider whether the absence of evidence can justify lack of taking action.
References (pdf)
You may also be interested in reading the following blogs:
Teapots and unicorns: absence of evidence is not evidence of absence
An invisible unicorn has been grazing in my office for a month… Prove me wrong
Don’t confuse “no evidence of a difference” with “evidence of no difference”
A beginner’s guide to interpreting odds ratios, confidence intervals and p-values
Confidence intervals should be reported
No Comments on ‘No evidence of effect’ versus ‘evidence of no effect’: how do they differ?
Tanks for sharing this information. I really like your blog post very much.
4th July 2023 at 11:03 amI am worndering to find such an informative content
4th July 2023 at 11:01 am